- 자동번역기로 번역된 논문입니다.
Globally and Locally Consistent Image Completion
SATOSHI IIZUKA, Waseda UniversityEDGAR SIMO-SERRA, Waseda UniversityHIROSHI ISHIKAWA, Waseda UniversityFig. 1"
"전 세계적으로 그리고 지역적으로 일관성 있는 이미지 완성사토시 IIZUKA, 와세다 대학 SIMO-SERRA, 와세다 대학 ISHIROSHI ISHIKAWA, 와세다 대학 Fig.1"
Image completion results by our approach. The masked area is shown in white
"이미지 완성 결과, 복면 영역은 흰색으로 표시됨"
" Our approach can generate novel fragments that are not presentelsewhere in the image, such as needed for completing faces; this is not possible with patch-based methods. Photographs courtesy of Michael D Beckwith(CC0), Mon Mer (Public Domain), davidgsteadman (Public Domain), and Owen Lucas (Public Domain)"
"우리의 접근방식은 얼굴 완성에 필요한 것과 같이 이미지의 다른 곳에 존재하지 않는 새로운 조각들을 생성할 수 있다; 이것은 패치 기반 방법으로는 불가능하다.사진 제공 마이클 D 벡위드(CC0), 몬 머(공공 도메인), 데이비드그스테드먼(공공 도메인), 오웬 루카스(공공 도메인)"
"We present a novel approach for image completion that results in imagesthat are both locally and globally consistent. With a fully-convolutionalneural network, we can complete images of arbitrary resolutions by
ling-in missing regions of any shape"
우리는 지역적으로나 세계적으로 일관된 이미지를 만들어 내는 새로운 이미지 완성 접근법을 제시한다.완전 컨볼루션 신경망을 사용하면 임의 해상도의 이미지를 lling-in 결측 영역으로 완성할 수 있습니다.
" To train this image completion network tobe consistent, we use global and local context discriminators that are trainedto distinguish real images from completed ones. The global discriminatorlooks at the entire image to assess if it is coherent as a whole, while the localdiscriminator looks only at a small area centered at the completed region toensure the local consistency of the generated patches"
"이 이미지 완성 네트워크를 일관성있게 훈련하기 위해 실제 이미지와 완성된 이미지를 구별하도록 훈련된 글로벌 및 로컬 컨텍스트 판별기를 사용합니다.글로벌 판별기는 전체 이미지를 보고 전체 일관성이 있는지 평가하는 반면, 로컬 판별기는 생성된 패치의 로컬 일관성을 보장하기 위해 완료된 영역을 중심으로 작은 영역만 본다."
" The image completionnetwork is then trained to fool the both context discriminator networks,which requires it to generate images that are indistinguishable from real oneswith regard to overall consistency as well as in details. We show that ourapproach can be used to complete a wide variety of scenes"
"그런 다음 이미지 완성 네트워크는 컨텍스트 판별기 네트워크를 모두 속이도록 훈련되며, 이는 전체적인 일관성과 세부 사항과 관련하여 실제 이미지와 구별할 수 없는 이미지를 생성해야 합니다.우리는 우리의 접근이 다양한 장면들을 완성하는데 사용될 수 있다는 것을 보여준다."
" Furthermore, incontrast with the patch-based approaches such as PatchMatch, our approachcan generate fragments that do not appear elsewhere in the image, whichallows us to naturally complete the images of objects with familiar andhighly speci(cid:27)c structures, such as faces. CCS Concepts: • Computing methodologies → Image processing; Neu-ral networks;This work was partially supported by JST ACT-I Grant Number JPMJPR16U3 and JSTCREST Grant Number JPMJCR14D1"
"또한 패치 기반 접근 방식과는 달리, 우리의 접근 방식은 이미지의 다른 곳에 나타나지 않는 단편을 생성할 수 있으며, 이는 얼굴과 같은 친숙하고 높은 speci (cid : 27)c 구조를 가진 물체의 이미지를 자연스럽게 완성할 수 있게 합니다.CCS 개념 : • 컴퓨팅 방법론 ; 중립 네트워크;이 작업은 JST ACT-I Grant Number JPMJPR16U3 및 JSTCREST Grant Number JPMJCR14D1에 의해 부분적으로 지원되었습니다."
Globally andLocally Consistent Image Completion. ACM Trans
"전지구적, 논리적으로 일관된 이미지 완성 ACM 트랜스"
" Graph. 36, 4, Article 107(July 2017), 14 pages"
"그래프 36, 4조, 제107조(2017년 7월), 14페이지"
INTRODUCTION
Image completion is a technique that allows (cid:27)lling-in target regionswith alternative contents. This allows removing unwanted objectsor generating occluded regions for image-based 3D reconstruction
org/10.1145/3072959.3073659
1Image 완료는 대체 콘텐츠를 가진 대상 영역을 (cid:27)lling-in할 수 있는 기술이다.이를 통해 원하지 않는 객체를 제거하거나 이미지 기반 3D 재구성을 위해 폐색된 영역을 생성할 수 있습니다
"Although many approaches have been proposed for image comple-tion, such as patch-based image synthesis [Barnes et al. 2009; Darabiet al, 2012; Huang et al. 2014; Simakov et al , 2012; Huang et al. 2014; Simakov et al. " 2008; Wexler et al. 2007],
it remains a challenging problem because it often requires high-levelrecognition of scenes"
패치 기반 이미지 합성 [Barnes et al.]과 같은 이미지 컴플레이션을 위한 많은 접근법이 제안되었지만.
2008; Wexler 등.2007년, 그것은 종종 높은 수준의 장면 인식을 필요로 하기 때문에 여전히 도전적인 문제로 남아있다."
" Not only is it necessary to complete texturedpatterns, it is also important to understand the anatomy of the sceneand objects being completed. Based on this observation, in this workwe consider both the local continuity and the global composition ofthe scene, in a single framework for image completion"
"질감 패턴을 완성하는 것뿐만 아니라, 완성되는 장면과 물체의 해부학을 이해하는 것도 중요하다.이 관찰을 바탕으로, 본 연구에서는 영상 완성을 위한 하나의 프레임워크에서 장면의 로컬 연속성과 전역적 구성을 모두 고려한다."
"Our work builds upon the recently proposed Context Encoder(CE) approach [Pathak et al. 2016], which employs a Convolutional Neural Network (CNN) that is trained with an adversarial loss [Good-fellow et al 2014]. The CE approach was motivated by feature learn-ing, and did not fully describe how to handle arbitrary inpaintingmasks nor how to apply the approach to high resolution images"
"출판일 : 2017년 7월.이즈카 사토시, 시모 세라, 이시카와 뉴럴 네트워크(CNN)는 적의 손실로 훈련된다[Good-fellow et al 2014.]CE 접근법은 기능 학습에 의해 동기 부여되었으며 임의의 인페인팅 마스크를 다루는 방법 또는 고해상도 이미지에 접근 방법을 완전히 설명하지 않았습니다
" Ourproposed approach addresses these two points and further improvesthe visual quality of the results as we shall see. We leverage a fully convolutional network as the basis of ourapproach, and propose a novel architecture that results in bothlocally and globally consistent natural image completion"
"본 논문에서 제안한 접근방식은 이 두 가지 점을 다루고, 앞으로 보겠지만 결과의 시각적 질을 더욱 향상시킨다.우리는 우리의 접근의 기초로서 완전한 컨볼루션 네트워크를 활용하고, 지역적으로나 세계적으로 일관된 자연 이미지 완성을 초래하는 새로운 아키텍처를 제안한다."
" Our ar-chitecture is composed of three networks: a completion network, aglobal context discriminator, and a local context discriminator. Thecompletion network is fully convolutional and used to completethe image, while both the global and the local context discrimina-tors are auxiliary networks used exclusively for training"
"우리의 아르-치텍처는 완성 네트워크, 세계적 컨텍스트 판별기, 그리고 지역 컨텍스트 판별기의 세 가지 네트워크로 구성된다.완료 네트워크는 완전히 컨볼루션적이며 이미지를 완성하는 데 사용되는 반면 글로벌 및 로컬 컨텍스트 디스크리미나-터는 훈련 전용으로 사용되는 보조 네트워크다."
" Thesediscriminators are used to determine whether or not an image hasbeen completed consistently. The global discriminator takes thefull image as input to recognize global consistency of the scene,while the local discriminator looks only at a small region aroundthe completed area in order to judge the quality of more detailedappearance"
"이러한 판별기는 이미지가 일관되게 완료되었는지 여부를 결정하는 데 사용됩니다.글로벌 판별기는 장면의 전체적인 일관성을 인식하기 위한 입력으로 전체 이미지를 취하는 반면, 로컬 판별기는 보다 상세한 외관의 품질을 판단하기 위해 완성된 영역 주변의 작은 영역만을 본다."
" During each training iteration, the discriminators areupdated (cid:27)rst so that they correctly distinguish between real andcompleted training images. Afterwards, the completion networkis updated so that it (cid:27)lls the missing area well enough to fool thecontext discriminator networks"
"각 훈련 반복 동안, 판별기는 실제와 완성된 훈련 이미지를 정확하게 구별하기 위해 업데이트된다(cid:27).이후, 완료 네트워크는 상황 식별자 네트워크를 속일 수 있을 만큼 누락된 영역을 잘 표시하도록 업데이트된다(cid:27)."
" As shown in Fig. 1, using both thelocal and the global context discriminators is critical for obtainingrealistic image completion"
"그림에서 보듯이.1, 지역적 상황과 글로벌 상황의 판별자를 모두 사용하는 것은 현실적인 이미지 완성을 위해 매우 중요하다."
"We evaluate and compare our approach with existing methods ona large variety of scenes. We also show results on more challengingspeci(cid:27)c tasks, such as face completion, in which our approach cangenerate image fragments of objects such as eyes, noses, or mouthsto realistically complete the faces"
"우리는 우리의 접근법을 다양한 장면에서 기존의 방법과 평가하고 비교한다.또한 얼굴 완성과 같은 좀 더 도전적인(cid:27)c 과제에 대한 결과를 보여 주는데, 이 과제에서 우리의 접근방식은 눈, 코, 입과 같은 물체의 이미지 파편을 현실적으로 완성시킬 수 있다."
" We evaluate the naturalness of thischallenging face completion with a user study, where the di(cid:29)erencebetween our results and real faces is indiscernible 77% of the time. In summary, in this paper we present:• a high performance network model that can complete arbitrarymissing regions,• a globally and locally consistent adversarial training approachfor image completion, and• results of applying our approach to speci(cid:27)c datasets for morechallenging image completion"
"우리는 이 도전적인 얼굴 완성의 자연성을 사용자 연구로 평가하는데, 여기서 우리의 결과와 실제 얼굴 사이의 di(cid:29)erence는 77%의 시간 동안 구별할 수 없다.본 논문에서는 영상 완성을 위한 전 세계적으로 그리고 국지적으로 일관된 적의 훈련 방법을 제시하고, 영상 완성을 위한 데이터 세트를 speci(cid:27)c에 적용하여 보다 도전적인 영상 완성을 위한 방법을 제시한다."
2 RELATED WORK
A variety of different approaches have been proposed for the imagecompletion task. One of the more traditional approaches is thatof diffusion-based image synthesis
2 영상완성 과제를 위해 다양한 different 접근법이 제안되었다.보다 전통적인 접근 방법 중 하나는 diffusion 기반 이미지 합성이다.
" This technique propagates thelocal image appearance around the target holes to (cid:27)ll them in. Forexample, the propagation can be performed based on the isophotedirection (cid:27)eld [Ballester et al"
이 기술은 대상 구멍 주변의 로컬 이미지 모양을 (cid : 27)로 전파합니다.예시적 동위원소 방향(cid:27)eld[Ballester et al]에 기초하여 전파를 수행할 수 있다
" 2001; Bertalmio et al. 2000], or globalimage statistics based on the histograms of local features [Levinet al"
2001; Bertalmio 등.2000) 또는 국소적 특징의 히스토그램에 기초한 글로벌 이미지 통계[Levinet al.
" 2003]. However, di(cid:29)usion-based approaches, in general, canonly (cid:27)ll small or narrow holes, such as scratches found commonlyin old photographs"
2003].그러나 일반적으로 di(cid:29)usion 기반 접근방식은 오래된 사진에서 흔히 발견되는 긁힘과 같은 작거나 좁은 구멍만 있을 수 있다.
"In contrast to the di(cid:29)usion-based techniques, patch-based ap-proaches have been able to perform more complicated image com-pletion that can (cid:27)ll large holes in natural images. Patch-based imageACM Transactions on Graphics, Vol"
"di(cid:29)usion 기반 기법과는 달리, 패치 기반 ap-proaches는 자연 이미지에서 큰 구멍을 낼 수 있는 더 복잡한 이미지 콤플레이션을 수행할 수 있었다.그래픽에 대한 패치 기반 이미지ACM 트랜잭션, Vol"
Table 1
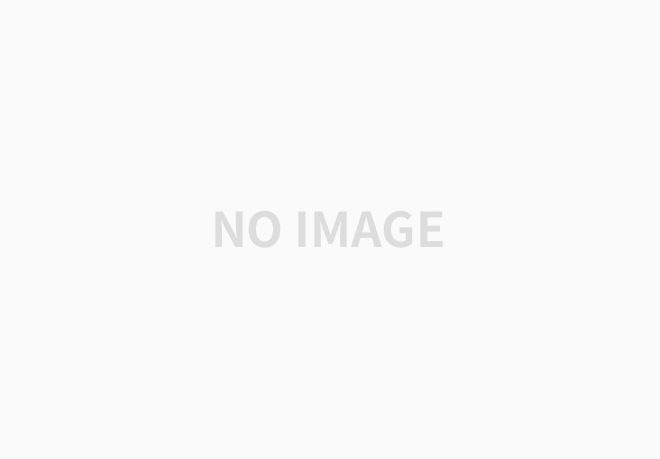
"Patch-based Context encoder OursImage sizeLocal ConsistencySemanticsNovel objectsAnyYesNoNoFixedNoYesYesAnyYesYesYescompletion was (cid:27)rst proposed for texture synthesis [Efros and Le-ung 1999; Efros and Freeman 2001], in which texture patches aresampled from a source image and then pasted into a target image. This was later extended with image stitching [Kwatra et al"
패치 기반 Context 인코더 OursImage 크기 지역 일관성시맨틱스노벨 객체AnyYesNoFixedNoYesYesYesecompletion은 텍스처 합성을 위해 제안된 (cid:27)rst [Efros and Le-ung 1999; Efros and Freeman 2001]로 텍스처 패치를 소스 이미지에서 샘플링한 다음 대상 이미지에 붙여넣었다.이것은 나중에 이미지 스티칭으로 확장되었다
[Kwatra et al. 2003]with graph cuts and texture generation [Kwatra et al. 2005] based onenergy optimization
2003] 그래프 절단 및 텍스처 생성[Kwatra et al. 2005] 기반 에너지 최적화
" For image completion, several modi(cid:27)cationssuch as optimal patch search have been proposed [Bertalmio et al. 2003; Criminisi et al"
영상 완성을 위해 최적의 패치 검색과 같은 여러 가지 modi (cid : 27) 양이 제안되었습니다 [Bertalmio et al.2003; Criminisi et al. 2004; Drori et al. 2003]
" In particular, Wexler etal. [2007] and Simakov et al" [2008] proposed a global-optimization-based method that can obtain more consistent (cid:27)lls. These techniqueswere later accelerated by a randomized patch search algorithm calledPatchMatch [Barnes et al
"특히, Wexler etal. [2007]과 Simakov et al." [2008]은보다 일관된 (cid : 27) lls를 얻을 수 있는 글로벌 최적화 기반 방법을 제안했습니다.이러한 기법은 나중에 PatchMatch[Barnes et al]라는 랜덤화된 패치 검색 알고리즘에 의해 가속화되었다.
" 2009, 2010], which allows for real-timehigh-level image editing of images. Darabi et al"
"2009, 2010, )은 이미지의 실시간 고수준 이미지 편집을 가능하게 한다."
" [2012] demonstratedimproved image completion by integrating image gradients into thedistance metric between patches. However, these methods dependon low-level features such as the sum of squared di(cid:29)erences of patchpixel values, which are not e(cid:29)ective to (cid:27)ll in holes on complicatedstructures"
[2012]는 이미지 구배를 패치 간의 거리 메트릭에 통합하여 향상된 이미지 완성을 입증했습니다.그러나 이러한 방법은 복잡한 구조의 구멍에서 (cid : 27)ll에 영향을 미치지 않는 패치 픽셀 값의 제곱 di (cid : 29)erences 합과 같은 낮은 수준의 특징에 의존합니다.
" Furthermore, they are unable to generate novel objectsnot found in the source image, unlike our approach. To tackle the problem of generating large missing regions of struc-tured scenes, there are some approaches that use structure guidance,which are generally speci(cid:27)ed manually, to preserve important un-derlying structures"
"또한, 그들은 우리의 접근 방식과는 달리 소스 이미지에서 발견되지 않는 새로운 물체를 생성할 수 없다.구조가 없는 장면의 큰 누락 영역을 생성하는 문제를 해결하기 위해 일반적으로 스펙 (cid : 27)을 수동으로 사용하여 중요한 비-델링 구조를 보존하는 몇 가지 접근법이 있습니다."
" This can be done by specifying points of inter-est [Drori et al. 2003], lines or curves [Barnes et al"
"이것은 inter-est [Drori et al.]의 점을 지정하여 수행할 수 있습니다.2003), 선 또는 곡선[Barnes et al."
" 2009; Sun et al. 2005], and perspective distortion [Pavić et al"
"2009; Sun et al. 2005), 원근법 왜곡 [Pavi et al."
2006]. Approaches forautomatic estimation of the scene structure have also been proposed:utilizing the tensor-voting algorithm to smoothly connect curvesacross holes [Jia and Tang 2003]; exploiting structure-based priorityfor patch ordering [Criminisi et al
"2006.또한, 텐서-투표 알고리즘을 이용하여 곡선형 홀[Jia and Tang 2003]을 원활하게 연결하고, 패치 오더링을 위한 구조 기반 우선순위를 이용하여 장면 구조를 자동 추정하는 방법이 제안되었다."
" 2004], tile-based search spaceconstraints [Kopf et al. 2012], statistics of patch o(cid:29)sets [He and Sun2012], and regularity in perspective planar surfaces [Huang et al"
"2004), 타일 기반 검색 공간 제약 [Kopf et al.2012), 패치 o(cid:29)의 통계 [He and Sun2012] 및 원근법 평면 표면의 규칙성 [Huang et al]"
2014]. These approaches improve the quality of the image comple-tion by preserving important structures
2014.]이러한 접근방식은 중요한 구조를 보존함으로써 이미지 컴플레이션의 품질을 향상시킨다
" However, such guidancesare based on the heuristic constraints of speci(cid:27)c types of scenes andthus are limited to speci(cid:27)c structures. The obvious limitation of most existing patch-based approaches isthat the synthesized texture only comes from the input image"
"그러나 이러한 지도는 speci(cid:27)c 유형의 장면과thus의 휴리스틱 제약에 기초하고 있으며, speci(cid:27)c 구조에 한정되어 있다.대부분의 기존 패치 기반 접근법의 명백한 한계는 합성된 텍스처가 입력 이미지에서만 나온다는 것입니다"
Thisis a problem when a convincing completion requires textures thatare not found in the input image. Hays and Efros [2007] proposedan image completion method using a large database of images
이것은 설득력 있는 완성이 입력 이미지에서 찾을 수 없는 텍스처를 필요로 할 때 문제가 된다.대규모 이미지 데이터베이스를 이용한 Hays and Efros [2007] 제안 영상 완성 방법
TheyGlobally and Locally Consistent Image Completion •107:3Global DiscriminatorImage + MaskDilated Conv. Completion NetworkOutputFig
그들은 전지구적 및 지역적으로 일관성 있는 이미지 완성 •107:3글로벌 판별자 이미지 + 마스크딜레이티드 콘브.완료 네트워크 출력화
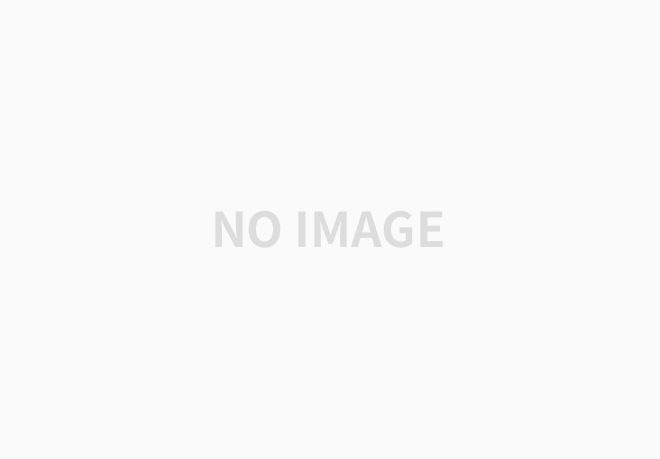
2. Overview of our architecture for learning image completion
2. 영상완성 학습을 위한 우리 건축의 개요
" It consists of a completion network and two auxiliary context discriminator networks thatare used only for training the completion network and are not used during the testing. The global discriminator network takes the entire image as input, whilethe local discriminator network takes only a small region around the completed area as input"
완료 네트워크와 완료 네트워크 훈련에만 사용되며 테스트 중에 사용되지 않는 두 개의 보조 컨텍스트 판별기 네트워크로 구성됩니다.글로벌 판별 네트워크는 전체 이미지를 입력으로 취하고 로컬 판별 네트워크는 입력으로 완료된 영역 주변의 작은 영역만 사용합니다
" Both discriminator networks are trained to determine if animage is real or completed by the completion network, while the completion network is trained to fool both discriminator networks. Local DiscriminatorReal or Fake(cid:27)rst search for the image most similar to the input in the database,and then complete the image by cutting the corresponding regionsfrom the matched image and pasting them into the holes"
"두 판별기 네트워크는 모두 영상이 실제인지 또는 완료 네트워크에 의해 완료되었는지를 결정하도록 훈련되는 반면, 완료 네트워크는 두 판별기 네트워크를 모두 속이도록 훈련된다.로컬 판별기 Real 또는 Fake (cid : 27)rst는 데이터베이스의 입력과 가장 유사한 이미지를 검색한 다음 일치하는 이미지에서 해당 영역을 잘라 구멍에 붙여 이미지를 완료합니다."
" However,this assumes that the database contains an image similar to theinput image, which may not be the case. This was also extended tothe particular case in which images of exactly the same scene areincluded in the database of images [Whyte et al"
"그러나 이것은 데이터베이스에 입력 이미지와 유사한 이미지가 포함되어 있다고 가정하며, 그렇지 않을 수도 있다.이것은 또한 정확히 같은 장면의 이미지가 이미지 데이터베이스에 포함된 특정 사례로 확장되었습니다 [Whyte et al."
" 2009]. However,the assumption that the exact same scene is included limits theapplicability greatly in comparison to general approaches"
"2009].그러나, 정확히 같은 장면이 포함되어 있다는 가정은 일반적인 접근방식에 비해 적용 가능성을 크게 제한한다."
Completion of human faces has also received attention as a par-ticular application of inpainting. Mohammed et al
인간의 얼굴 완성도는 또한 인페인팅의 파티큘러 적용으로 주목을 받았다.모하메드 외
[2009] build apatch library using a dataset of faces and propose a global and lo-cal parametric model for face completion. Deng et al
[2009] 얼굴 데이터 세트를 사용하여 패치 라이브러리를 구축하고 얼굴 완성을 위한 전역 및 lo-cal 파라 메트릭 모델을 제안합니다.덩 외
" [2011] use aspectral-graph-based algorithm for face image repairing. However,these approaches require aligned images for learning patches, anddo not generalize to the arbitrary inpainting problem"
[2011] 얼굴 이미지 복구를 위해 종횡 그래프 기반 알고리즘을 사용합니다.그러나 이러한 접근 방식은 패치를 학습하기 위해 정렬된 이미지를 필요로 하며 임의의 인페인팅 문제에 일반화하지 않는다.
"Convolutional Neural Networks (CNNs) have also been usedfor image completion. Initially, CNN-based image inpainting ap-proaches were limited to very small and thin masks [Köhler et al"
컨볼루션 신경망(CNN)은 영상 완성에도 사용되었다.처음에 CNN 기반 이미지 인페인팅 ap-proaches는 매우 작고 얇은 마스크로 제한되었다 [Köhler et al.
2014; Ren et al. 2015; Xie et al
2014; Ren et al. 2015; Xie et al.
2012]. Similar approaches have alsobeen applied to MRI and PET images for completing missing data [Liet al
2012.MRI와 PET 영상에서도 결측 데이터를 완성하기 위해 유사한 접근법이 적용되었다[Liet al]
" 2014]. More recently, and concurrently to this work, Yang etal"
"2014) 보다 최근에, 그리고 동시에 이 작품에 양에탈"
" [2017] also proposed a CNN based optimization approach forinpainting. However, unlike our approach, this has an increasedcomputation time due to having to optimize for every image"
"[2017] 또한 CNN 기반 최적화 접근법을 제안했습니다.하지만, 우리의 접근 방식과는 달리, 이것은 모든 이미지에 최적화되어야 하기 때문에 계산 시간이 증가한다"
"We build upon the recently proposed Context Encoder (CE) [Pathaket al. 2016], that extended CNN-based inpainting to large masks,and proposed a context encoder to learn features by inpainting,based on Generative Adversarial Networks (GAN) [Goodfellow et al"
"우리는 최근에 제안된 Context Encoder (CE) [Pathaket al.2016년, CNN 기반 인페인팅을 대형 마스크로 확장하고 GAN(Generative Adversarial Networks) [Goodfellow et al."
2014]. The original purpose of GAN is to train generative modelsusing convolutional neural networks
2014.]GAN의 원래 목적은 컨볼루션 신경망을 이용한 생성 모델을 훈련시키는 것이다.
" These generator networks aretrained by using an auxiliary network, called discriminator, whichserves to distinguish whether an image is generated by a networkor is real. The generator network is trained to fool the discriminatornetwork, while the discriminator network is updated in parallel"
"이러한 생성기 네트워크는 식별자라고 불리는 보조 네트워크를 사용하여 훈련되며, 이는 네트워크에 의해 이미지가 생성되는지 여부를 구별하는 데 도움이 된다.발전기 네트워크는 판별기 네트워크를 속이도록 훈련되고, 판별기 네트워크는 병렬로 업데이트된다"
" Byusing a Mean Squared Error (MSE) loss in combination with a GANloss, Pathak et al. [2016] were able to train an inpainting networkto complete a 64 × 64 pixel area in the center of 128 × 128 pixelimages, avoiding the blurring common with using only MSE losses"
"평균 제곱 오차(MSE) 손실을 GANloss, Pathak 등과 결합하여 사용함으로써.[2016]은 128 픽셀 이미지의 중심에 64 64 픽셀 영역을 완료하기 위해 인페인팅 네트워크를 훈련할 수 있었으며 MSE 손실만 사용하는 것과 공통되는 흐릿한 현상을 피할 수 있었습니다."
"We extend their work to handle arbitrary resolutions by using afully convolutional network, and signi(cid:27)cantly improve the visualquality by employing both a global and local discriminator. One of the main issues of GAN is the instability during learning,which has led to numerous research on the topic [Radford et al"
"우리는 완전한 컨볼루션 네트워크를 이용하여 임의의 해상도를 처리하기 위해 작업을 확장하고, 시그니(cid:27)는 글로벌 및 로컬 판별기를 모두 사용하여 시각 품질을 향상시킬 수 있다.GAN의 주요 문제 중 하나는 학습 중 불안정성으로 인해 [Radford et al] 주제에 대한 수많은 연구가 이루어졌습니다."
2016;Salimans et al. 2016]
2016; Salimans et al. 2016]
" We avoid this issue by not training purelygenerative models and tuning the learning process to prioritizestability. Additionally, we have heavily optimized the architectureand the training procedure speci(cid:27)cally for the image completionproblem"
"우리는 순수 생성 모델을 훈련시키지 않고 학습 과정을 우선순위로 조정함으로써 이 문제를 피한다.또한, 영상 완성 문제를 위해 아키텍처와 훈련 절차 스펙(cid:27)을 크게 최적화하였다."
" In particular, we do not use a single discriminator but two:a global discriminator network and a local discriminator network. As we show, this proves critical in obtaining semantically and locallycoherent image completion results"
"특히, 우리는 단일 판별기를 사용하지 않고 두 가지, 즉 글로벌 판별기 네트워크와 로컬 판별기 네트워크를 사용합니다.우리가 보여주듯이, 이것은 의미적이고 지역적으로 일관성 있는 이미지 완성 결과를 얻는 데 매우 중요하다."
Our approach can overcome the limitations of the existing ap-proaches and realistically complete diverse scenes. A high-levelcomparison of di(cid:29)erent approaches can be seen in Table 1
우리의 접근은 기존의 ap-proaches의 한계를 극복하고 현실적으로 다양한 장면들을 완성할 수 있다.di(cid:29)erent 접근법의 높은 수준 비교는 표 1에서 볼 수 있다.
" On onehand, the patch-based approaches [Barnes et al. 2009, 2010; Darabiet al"
"한편, 패치 기반 접근법 [Barnes et al. 2009, 2010; Darabiet al."
2012; Huang et al. 2014; Wexler et al
2012; Huang et al. 2014; Wexler et al.
" 2007] show high qualityreconstructions for arbitrary image sizes and masks; however, theyare unable to provide novel image fragments not found elsewherein the image nor have a high level semantic understanding of theimage: they only local at similarity on a local patch level. On theother hand, the context encoder-based approach [Pathak et al"
"2007년 에서는 임의의 이미지 크기와 마스크에 대한 고품질의 재구성을 보여 주지만 이미지의 다른 곳에서는 발견되지 않은 새로운 이미지 조각을 제공할 수 없으며 이미지에 대한 높은 의미 론적 이해를 가질 수 없습니다. 로컬 패치 레벨에서 유사하게만 로컬입니다.한편, 컨텍스트 인코더 기반 접근법[Pathak et al."
" 2016]can generate novel objects, but are limited to (cid:27)xed low resolutionimages. Furthermore, the approach can lack local consistency asthe continuity of the completed region with the surrounding area isnot taken into account"
"2016]은 새로운 객체를 생성할 수 있지만 (cid : 27)xed low resolution 이미지로 제한됩니다.또한, 주변지역과의 연속성을 고려하지 않으므로, 접근방식은 국부적 일관성이 결여될 수 있다."
" Our approach can deal with arbitrary imagesizes and masks, while being consistent with the image and able togenerate novel objects. ACM Transactions on Graphics, Vol"
"우리의 접근방식은 임의의 이미지화와 마스크를 다룰 수 있는 동시에 이미지와 일치하고 새로운 물체를 생성할 수 있다.그래픽에 대한 ACM 거래, Vol"
" 36, No. 4, Article 107"
"36, 제4조, 제107조"
" Publication date: July 2017. 107:4• Satoshi Iizuka, Edgar Simo-Serra, and Hiroshi Ishikawa3 APPROACHOur approach is based on deep convolutional neural networkstrained for the image completion task"
"출판일 : 2017년 7월.이즈카 사토시, 시모세라, 이시카와 히로시의 접근방식은 영상완료 과제를 위해 훈련된 심층 컨볼루션 신경망을 기반으로 한다."
" A single completion net-work is used for the image completion. Two additional networks,the global and the local context discriminator networks, are used inorder to train this network to realistically complete images"
이미지 완성을 위해 단일 완성 네트워크가 사용된다.글로벌 및 로컬 컨텍스트 판별 네트워크인 두 개의 추가 네트워크가 이 네트워크를 현실적으로 완료하도록 훈련하는 데 순서대로 사용됩니다
" Duringthe training, the discriminator networks are trained to determinewhether or not an image has been completed, while the comple-tion network is trained to fool them. Only by training all the threenetworks together is it possible for the completion network to realis-tically complete a diversity of images"
"훈련 중에, 판별기 네트워크는 이미지가 완료되었는지 여부를 결정하도록 훈련되고, 보완 네트워크는 그들을 속이도록 훈련된다.세 개의 네트워크 모두를 함께 훈련해야만 완성 네트워크가 다양한 이미지를 현실적으로 완성할 수 있다."
An overview of this approachcan be seen in Fig. 2.3
이 접근법의 개요는 그림 2.3에서 볼 수 있다.
1 Convolutional Neural NetworksOur approach is based on Convolutional Neural Networks [Fukushima1988; LeCun et al. 1989]
1 Convolutional Neural Networks우리의 접근방식은 Convolutional Neural Networks [Fukushima1988; LeCun 등]에 기초한다.1989년]
" These are a special variant of neural net-work based on using convolution operators that conserve the spatialstructure of the input, generally consisting of images. These net-works are formed by layers in which a bank of (cid:27)lters is convolutedwith the input map to produce an output map which is furtherprocessed with a non-linear activation function, most often theRecti(cid:27)ed Linear Unit (ReLU), de(cid:27)ned as σ (·) = max (·, 0) [Nair andHinton 2010]"
"이들은 일반적으로 이미지로 구성된 입력의 공간 구조를 보존하는 컨볼루션 연산자를 사용하는 것에 기반한 신경망 작업의 특별한 변형입니다.이러한 네트웍은 (cid:27)lters의 뱅크가 입력 맵과 컨볼루션되어 비선형 활성화 함수로 추가 처리되는 출력 맵을 생성하는 레이어에 의해 형성된다. 가장 자주 Recti(cid:27)ed Linear Unit (ReLU), de(cid:27) = max (········· 0) [Nair and Hinton 2010]"
"Instead of using only the standard convolutional layers, we alsoemploy a variant called the dilated convolution layers [Yu andKoltun 2016], which allow increasing the area each layer can useas input. This is done without increasing the number of learnableweights by spreading the convolution kernel across the input map"
"표준 컨볼루션 계층만을 사용하는 대신, 우리는 또한 각 계층이 입력으로 사용할 수 있는 면적을 증가시킬 수 있는 확장된 컨볼루션 계층[Yu and Koltun 2016]이라는 변형을 채용한다.이것은 입력 맵에 콘볼루션 커널을 퍼뜨려 학습 가능한 가중치의 수를 증가시키지 않고 수행됩니다"
"More speci(cid:27)cally, if one 2D layer is a C-channel h × w map andthe next layer is a C ′-channel h′ × w ′ map, the dilated convolutionoperator can be written for each pixel as:b +*. ,kh − 1k ′hXi =−k ′hk ′wXj =−k ′wWk ′h+i ,k ′w +j xu +ηi ,v +η j,k ′w =kw − 12,+//-,(1)yu ,v = σk ′h =2where kw and kh are the kernel width and height (odd numbers),respectively, η is the dilation factor, xu ,v ∈ RC and yu ,v ∈ RC ′arethe pixel component of the input and the output of the layer, σ (·) isa component-wise non-linear transfer function, Ws ,t are C ′-by-Cmatrices of the kernel, and b ∈ RC ′is the layer bias vector"
"더 많은 speci (cid : 27)cally, 하나의 2D 레이어가 C- 채널 h w 맵이고 다음 레이어가 C- 채널 h w 맵인 경우, 확장된 컨볼루션 연산자는 각 픽셀에 대해 다음과 같이 작성 될 수 있습니다 : b + *.,kh 1k hXi = k k wXj = k k wwk h+i ,k w +j xu + i ,v + j,k =kw = 12,+/-, (1)yu ,v = kw = kw = kw = 2 kw = kw 와 kh 는 kernel 폭과 높이이다. (이상수)는 각각 입력의 확장 계수, xu,v RC 및 yu,v RC RC arethe 픽셀 성분, 층의 출력, (·) 성분-방향 비선형 전달 함수, Ws,t는 커널의 C by-Cmatrices이고, b RC는 층 바이어스이다. 벡터"
Withη = 1 the equation becomes the standard convolution operation. These networks are then trained to minimize a loss function withback-propagation [Rumelhart et al
상기 방정식은 표준 컨볼루션 연산이 되는 = 1이다.그런 다음 이러한 네트워크는 역전파로 손실 함수를 최소화하도록 훈련된다 [Rumelhart et al.
" 1986], and are trained by usingdatasets which consist of input and output pairs. The loss functionusually tries to minimize the distance between the network outputand the corresponding output pair in the dataset"
"1986년), 입력 및 출력 쌍으로 구성된 데이터 세트를 사용하여 교육을 받는다.손실은 데이터 세트에서 네트워크 출력과 해당 출력 쌍 사이의 거리를 최소화하기 위해 일반적으로 시도됩니다"
3.2 Completion NetworkThe completion network is based on a fully convolutional network. An overview of the network model architecture can be seen inTable 2
3.2 완료 네트워크 완료 네트워크는 완전 컨볼루션 네트워크를 기반으로 한다.네트워크 모델 아키텍처의 개요는 InTable 2를 볼 수 있습니다.
" The input of the completion network is an RGB image witha binary channel that indicates the image completion mask (1 fora pixel to be completed), and the output is an RGB image. As weACM Transactions on Graphics, Vol"
"완성 네트워크의 입력은, 완성 마스크(1화소가 완성되는 것을 위한 화소)를 나타내는 이진 채널을 갖는 RGB 이미지이고, 출력은 RGB 이미지이다.그래픽에 대한 WeACM 트랜잭션으로서, Vol"
" 36, No. 4, Article 107"
"36, 제4조, 제107조"
Publication date: July 2017. Influencing regionHoleΩΩΩΩFig
"발행일 : 2017년 7월, 지역 영향 홀피그"
3. Importance of spatial support
3. 공간지원의 중요성
" In order to be able to complete largeregions, the spatial support used to compute an output pixel must includepixels outside of the hole. On the le(cid:28), the pixel p1 is computed from theinfluencing region in the spatial support Ω1, while the pixel p2 cannot becalculated since the supporting area Ω2 does not contain any informationoutside of the hole"
"더 큰 영역을 완료할 수 있으려면 출력 픽셀을 계산하는 데 사용되는 공간 지원에는 홀 외부의 픽셀이 포함되어야 합니다.le(cid:28)에서, 픽셀 p1은 공간 지지 (1) 내의 영향 영역으로부터 계산되는 반면, 픽셀 p2는 지지 영역 (2) 에 홀 외부의 어떠한 정보도 포함하지 않기 때문에 계산될 수 없다."
" However, on the right side, the spatial support is largerthan the hole, allowing the completion of the center pixels. do not wish any change in areas other than the completion regions,the output pixels outside of the completion regions are restored tothe input RGB values"
"그러나 오른쪽의 공간 지원은 구멍보다 커서 중앙 픽셀이 완성될 수 있다.완료 영역 이외의 영역에서 어떠한 변화도 원하지 않으며, 완료 영역 이외의 출력 픽셀은 입력 RGB 값으로 복원된다"
" The general architecture follows an encoder-decoder structure, which allows reducing the memory usage andcomputational time by initially decreasing the resolution beforefurther processing the image. Afterwards, the output is restoredto the original resolution using deconvolution layers [Long et al"
"일반적인 아키텍처는 인코더-디코더 구조를 따르며, 이 구조는 이미지를 처리하기 전에 해상도를 초기에 감소시킴으로써 메모리 사용량과 계산 시간을 줄일 수 있다.이후, 출력은 디컨볼루션 레이어들[Long et al]을 이용하여 원래의 해상도로 복원된다"
"2015], which consist of convolutional layers with fractional strides. Unlike other architectures that use many pooling layers to decreasethe resolution, our network model only decreases the resolutiontwice, using strided convolutions to 1/4 of the original size, which isimportant to generate non-blurred texture in the missing regions"
"2015년은 분수보행을 갖는 컨볼루션 층으로 구성되어 있다.해상도를 줄이기 위해 많은 풀링 레이어를 사용하는 다른 아키텍처와 달리, 우리 네트워크 모델은 스트라이드 컨볼루션을 원래 크기의 1/4로 사용하여 해상도를 두 번만 감소시키는데, 이는 누락된 영역에서 비블러드 텍스처를 생성하는 데 중요하다."
Dilated convolutional layers [Yu and Koltun 2016] are also usedin the mid-layers (Eq. (1) with η > 1)
확장된 컨볼루션 층[Yu and Koltun 2016]은 중간 층(Eq)에도 사용된다.(1) > 1)
" Dilated convolutions usekernels that are spread out, allowing to compute each output pixelwith a much larger input area, while still using the same amountof parameters and computational power. This is important for theimage completion task, as the context is critical for realism"
확장된 컨볼루션은 분산된 커널을 사용하여 훨씬 더 큰 입력 면적을 가진 각 출력 픽셀을 계산할 수 있지만 동일한 양의 매개 변수와 계산 전력을 사용합니다.이는 현실주의에 있어서 문맥이 중요하기 때문에 이미지 완성 작업에 중요하다.
" By usingdilated convolutions at lower resolutions, the model can e(cid:29)ectively“see” a larger area of the input image when computing each outputpixel than with standard convolutional layers. The resulting networkmodel computes each output pixel under the in(cid:30)uence of a 307 ×307-pixel region of the input image"
"낮은 해상도에서 확장된 컨볼루션을 사용함으로써, 모델은 표준 컨볼루션 레이어보다 각 출력 픽셀을 계산할 때 입력 이미지의 더 큰 영역을 e(cid:29)ectively"" 볼 수 있다.결과 네트워크 모델이 입력 영상의 307 픽셀 영역의 산:30uence(cid:30) 아래에 있는 각 출력 픽셀을 계산한다"
" Without using dilated convolutions,it would only use a 99 ×99-pixel region, not allowing the completionof holes larger than 99 × 99 pixels, as depicted in Fig. 3.3.3 Context DiscriminatorsA global context discriminator network and a local context discrim-inator network have the objective of discerning whether an imageis real or has been completed"
확장된 컨볼루션을 사용하지 않고는 99 픽셀 영역만 사용하며 그림에 묘사된 99 픽셀보다 큰 홀을 완성할 수 없습니다.3.3 문맥 판별자글로벌 문맥 판별자 네트워크와 로컬 문맥 판별자 네트워크는 이미지가 실제인지 또는 완료되었는지를 판별하는 목적을 가지고 있다
The networks are based on convolu-tional neural networks that compress the images into small featurevectors. Outputs of the networks are fused together by a concate-nation layer that predicts a continuous value corresponding to theprobability of the image being real
네트워크는 이미지를 작은 피쳐 벡터로 압축하는 공용 신경망을 기반으로 합니다.네트워크의 출력은 이미지가 실제일 확률에 대응하는 연속 값을 예측하는 연결 국가 계층에 의해 함께 융합된다
An overview of the networkscan be seen in Table 3. The global context discriminator takes as an input the entireimage rescaled to 256 × 256 pixels
네트워크 개요는 표 3에서 볼 수 있다.글로벌 컨텍스트 판별기는 256 픽셀로 재조정된 전체 이미지를 입력으로 사용합니다.
It consists of six convolutionallayers and a single fully-connected layer that outputs a single 1024-dimensional vector. All the convolutional layers employ a stride of2 × 2 pixels to decrease the image resolution while increasing theGlobally and Locally Consistent Image Completion •107:5Table 2
6 개의 컨볼루션 층과 단일 1024차원 벡터를 출력하는 단일 완전 연결된 층으로 구성됩니다.모든 컨볼루션 레이어는 2 픽셀의 보폭을 사용하여 이미지 해상도를 감소시키면서 전역 및 국부적으로 일관성있는 이미지 완료 •107 : 5Table 2
" Architecture of the image completion network. A(cid:28)er each con-volution layer, except the last one, there is a Rectified Linear Unit (ReLU)layer"
이미지 완료 네트워크의 아키텍처입니다.A (cid : 28)는 마지막 하나를 제외하고 각 콘볼루션 층에 정류된 선형 단위 (ReLU) 층이 있습니다.
" The output layer consists of a convolutional layer with a sigmoidfunction instead of a ReLU layer to normalize the output to the [0, 1] range. “Outputs” refers to the number of output channels for the output of thelayer"
"출력층은 [0, 1] 범위로 출력을 정규화하기 위해 RELU 층 대신 시그모이드 기능을 갖는 컨볼루션 층으로 구성된다.""출력""은 레이어 출력을 위한 출력 채널 수를 나타냅니다"
Table 3. Architectures of the discriminators used in our network model
표 3. 네트워크 모델에 사용되는 판별자의 구조
Fully-Connected (FC) layers refer to the standard neural network layers. Theoutput layer consists of a filly-connected layer with a sigmoid transfer layerthat outputs the probability that an input image came from real imagesrather than the completion network
완전 연결(FC) 층은 표준 신경망 층을 가리킨다.출력층은 입력 이미지가 완료 네트워크보다 실제 이미지로부터 나올 확률을 출력하는 시그모이드 전송 레이어를 갖는 필리 연결 레이어로 구성됩니다
(a) Local Discriminator(b) Global DiscriminatorTypeconv. conv
(a) 지역차별자(b) 글로벌차별자유형콘브
conv. conv
콘비
conv. conv
콘비
dilated conv. dilated conv
확장된 콘비
dilated conv. dilated conv
확장된 콘비
conv. conv
콘비
deconv. conv
콘브
deconv. conv
콘브
"outputKernel Dilation (η)Stride Outputs5 × 53 × 33 × 33 × 33 × 33 × 33 × 33 × 33 × 33 × 33 × 33 × 34 × 43 × 34 × 43 × 33 × 31111112481611111111 × 12 × 21 × 12 × 21 × 11 × 11 × 11 × 11 × 11 × 11 × 11 × 11/2 × 1/21 × 11/2 × 1/21 × 11 × 16412812825625625625625625625625625612812864323number of output (cid:27)lters. In contrast with the completion network,all convolutions use 5 × 5 kernels"
출력커널 확장()스트라이드 출력 5 53 33 33 33 33 33 33 33 33 33 33 33 33 33 33 33 33 33 33 33 33 33 43 34 43 43 43 33 33 33 33 33 311112481111111111111111 12 21 11 11 11 11 11 11 11 11 11 11 11 11 11 11 11 11/2 1/21 11/2 11/2 11 1/21 11 11 1/21 11 164128128256256256256255625625625625625625625612812864323 수의 출력 (cid : 27)lters.완료 네트워크와는 달리 모든 컨볼루션은 5 5개의 커널을 사용합니다
"The local context discriminator follows the same pattern, exceptthat the input is a 128 × 128-pixel image patch centered around thecompleted region. (Note that, at the training time, there is alwaysa single completed region"
"로컬 컨텍스트 판별기는 입력이 완료된 영역을 중심으로 128 픽셀 128 픽셀 이미지 패치라는 점을 제외하고는 동일한 패턴을 따릅니다.(참고로, 훈련 시간에는 항상 하나의 완성된 지역이 있다는 것이다."
" The trained completion network can,however, (cid:27)ll-in any number of holes at the same time. ) In the casethe image is not a completed image, a random patch of the imageis selected, as there is no completed region to center it on"
"훈련된 완료 네트워크는 동시에 모든 홀을 올인할 수 있습니다 (cid : 27).이미지가 완료된 이미지가 아닌 경우, 이미지의 랜덤 패치가 선택됩니다.이 이미지의 중심에 완료된 영역이 없기 때문입니다"
" As theinitial input resolution is half of the global discriminator, the (cid:27)rstlayer used in the global discriminator is not necessary. The outputis a 1024-dimensional vector representing the local context aroundthe completed region"
초기 입력 해상도가 글로벌 판별기의 절반이기 때문에 글로벌 판별기에 사용되는 (cid : 27)rstlayer는 필요하지 않습니다.출력은 완료된 영역 주변의 로컬 컨텍스트를 나타내는 1024차원 벡터입니다
"Finally, the outputs of the global and the local discriminatorsare concatenated together into a single 2048-dimensional vector,which is then processed by a single fully-connected layer, to outputa continuous value. A sigmoid transfer function is used so that thisvalue is in the [0, 1] range and represents the probability that theimage is real, rather than completed"
"마지막으로, 전역 및 로컬 판별기의 출력은 단일 2048차원 벡터로 함께 연결되며, 이 벡터는 단일 완전 연결된 레이어에 의해 처리되어 연속 값을 출력합니다.시그모이드 전달 함수는 이 값이 [0, 1] 범위에 있고 이미지가 완료되기보다는 실제일 확률을 나타내도록 사용된다."
"3.4 TrainingLet C (x, Mc ) denote the completion network in a functional form,with x the input image and Mc the completion region mask that isthe same size as the input image. The binary mask Mc takes the value1 inside regions to be (cid:27)lled-in and 0 elsewhere"
"3.4 TrainingLet C(x, Mc)는 기능적인 형태로 완료 네트워크를 나타내며, x는 입력 이미지, Mc는 입력 이미지와 동일한 크기인 완료 영역 마스크를 사용한다.이진 마스크 Mc는 값1 내부 영역(cid:27)을 lled-in으로, 다른 영역 0으로 취한다."
" As a preprocessing, Coverwrites the completion region of the training input image x by aconstant color, which is the mean pixel value of the training dataset,Type Kernel Stride OutputsType Kernel Stride Outputsconv. conv"
"전처리로서, 커버라이트는 트레이닝 데이터 세트의 평균 픽셀 값인 일정 컬러에 의해 트레이닝 입력 이미지 x의 완료 영역을 기록한다.콘브"
conv. conv
콘비
conv. 5 × 55 × 55 × 55 × 55 × 52 × 22 × 22 × 22 × 22 × 264128256512512FC--1024conv
5 55 55 55 55 55 55 52 22 22 22 22 22 22 22 264128256512512FC-1024conv
conv. conv
콘비
conv. conv
콘비
conv. 5 × 55 × 55 × 55 × 55 × 55 × 52 × 22 × 22 × 22 × 22 × 22 × 264128256512512512FC--1024(c) Concatenation layerType Kernel Stride Outputsconcat
대5 55 55 55 55 55 55 55 55 52 52 22 22 22 22 22 22 22 22 22 26412825651212FC--1024(c) 연결층형 커넬 스트라이드 출력스콘캣
"FC----20481before putting it into the network. Similarly, D (x, Md ) denotes thecombined context discriminators in a functional form"
"FC---20481이 네트워크에 넣기 전에마찬가지로 D(x, Md)는 결합 문맥 판별자를 기능적인 형태로 나타낸다."
"In order to train the network to complete the input image realisti-cally, two loss functions are jointly used: a weighted Mean SquaredError (MSE) loss for training stability, and a Generative AdversarialNetwork (GAN) [Goodfellow et al. 2014] loss to improve the realismof the results"
입력 이미지를 현실적으로 완료하기 위해 네트워크를 훈련하기 위해 두 가지 손실 함수가 공동으로 사용됩니다 : 훈련 안정성을 위한 가중 평균 SquardError (MSE) 손실 및 GAN (Generative AdversarialNetwork) [Goodfellow et al.2014] 결과의 사실성을 개선하기 위한 손실
" Using the mixture of the two loss functions allowsthe stable training of the high performance network model, andhas been used for image completion [Pathak et al. 2016], and con-currently with this work, for various image-to-image translationproblems [Isola et al"
"두 가지 손실 함수의 혼합물을 사용하여 고성능 네트워크 모델의 안정적인 훈련을 허용하고 이미지 완성에 사용되었습니다[Pathak et al.2016), 그리고 이 작품과 동시에 다양한 이미지 대 이미지 번역 문제를 위한 [Isola et al."
2017]. Training is done with backpropaga-tion [Rumelhart et al
"2017년, 백프로파가션[Rumelhart et al."
" 1986]. In order to stabilize the training, a weighted MSE loss consideringthe completion region mask is used [Pathak et al"
"1986].훈련을 안정화시키기 위해, 완료 영역 마스크를 고려한 가중된 MSE 손실이 사용된다[Pathak et al."
" 2016]. The MSEloss is de(cid:27)ned by:L(x, Mc ) = k Mc ⊙ (C (x, Mc ) − x ) k 2 ,(2)where ⊙ is the pixelwise multiplication and k · k is the Euclideannorm"
"2016].MSEloss는 다음과 같이 de(cid:27)ned by:L(x, Mc ) = k Mc (C(x, Mc ) ) x x ) k 2, (2) 여기서 는 픽셀 단위 곱셈이고 k · k는 유클리드어놈이다."
"The context discriminator networks also work as a kind of loss,sometimes called the GAN loss [Goodfellow et al. 2014]"
"상황 판별 네트워크는 또한 일종의 손실로 작용하며, 때로는 GAN 손실이라고도 합니다 [Goodfellow et al.2014]"
" This isthe crucial part of training in our approach, and involves turningthe standard optimization of a neural network into a min-max op-timization problem in which at each iteration the discriminatornetworks are jointly updated with the completion network. For ourcompletion and context discriminator networks, the optimizationbecomes:minCmaxDE[ log D (x, Md ) + log(1 − D (C (x, Mc ), Mc ) ] ,(3)where Md is a random mask, Mc is the input mask, and the expec-tation value is just the average over the training images x"
"이것은 우리의 접근방식에서 훈련의 중요한 부분이며, 신경망의 표준 최적화를 각 반복에서 판별망이 완성망과 공동으로 업데이트되는 최소 최대 최적화 문제로 바꾸는 것을 포함한다.우리의 완성 및 컨텍스트 판별 네트워크의 경우, 최적화는 다음과 같다:minCmaxDE[log D(x, Md) + log(1 D(C(x, Mc) ] , (3) 여기서 Md는 랜덤 마스크, Mc는 입력 마스크, expec-tation 값은 훈련 이미지 x에 대한 평균일 뿐이다."
"ACM Transactions on Graphics, Vol. 36, No"
"그래픽에 대한 ACM 거래, Vol. 36, No"
" 4, Article 107. Publication date: July 2017"
제107조 출판일: 2017년 7월
"107:6• Satoshi Iizuka, Edgar Simo-Serra, and Hiroshi IshikawaAlgorithm 1 Training procedure of the image completion network. 1: while iterations t < Tt r ain do2:3:Sample a minibatch of images x from training data"
"107:6 • Iizuka 사토시, Edgar Simo-Serra 및 이미지 완료 네트워크의 IshikawaAlgorithm 1 훈련 절차.1: 반복 t < Tt r은 do2:3:훈련 데이터에서 이미지 x의 미니배치를 표본으로 삼는다."
Generate masks Mc with random holes for each image x inthe minibatch. if t < TC then4:5:6:7:8:9:10:Update the completion network C with the weighted MSEloss (Eq
미니배치에서 각 이미지 x에 대해 랜덤 홀을 사용하여 마스크 Mc를 생성합니다.t < TC인 경우 4 : 5 : 6 : 7 : 8 : 9 : 10 : 가중치 MSEloss로 완료 네트워크 C를 업데이트하십시오 (Eq
" (2)) using (x, Mc ). elseGenerate masks Md with random holes for each image xin the minibatch"
"(2) (x, Mc)를 사용하는 것. 그렇지 않으면, Md는 미니배치의 각 이미지 xin에 대해 무작위 홀을 가지고 있다."
"Update the discriminators D with the binary cross entropyloss with both (C (x, Mc ), Mc ) and (x, Md ). if t > TC + TD thenUpdate the completion network C with the joint lossgradients (Eq"
"분별기 D를 이진 교차 엔트로필로스(C(x, Mc), Mc) 및 (x, Md)로 업데이트한다.t > TC + TD인 경우, 공동 손실분포로 완료 네트워크 C를 업데이트하십시오(Eq"
" (5)) using (x, Mc ), and D. end if11:12:13: end whileend ifBy combining the two loss functions, the optimization becomes:minCmaxDE[ L(x, Mc ) + α log D (x, Md )+ α log(1 − D (C (x, Mc ), Mc )) ],(4)where α is a weighing hyper parameter"
"(5)) (x, Mc) 및 D. end if 11:12:13: end interend if the two loss functions를 결합함으로써 최적화가 된다:minCmaxDE[L(x, Mc) + α log D(x, Md)+ α log(1 D(C(x, Mc)), Mc)), (4) 여기서 α는 가중 하이퍼 파라미터이다."
"During the course of the optimization, the completion and thediscriminator networks written here as C and D change, whichactually means that the weights and the biases of the networkschange. Let us denote the parameters of the completion networkC by θC "
최적화 과정에서 C와 D로 작성된 완료 및 판별자 네트워크가 변경됩니다. 이는 실제로 네트워크의 가중치와 편향이 변화 함을 의미합니다.완성 네트워크C의 파라미터를 C로 나타내자.
" In the standard stochastic gradient descent, the abovemin-max optimization then means that, for training C, we take thegradient of the loss function with respect to θC and update theparameters so that the value of the loss function decreases. Thegradient is:E[ ∇θC L(x, Mc ) + α ∇θC log(1 − D (C (x, Mc ), Mc )) ] "
"표준 확률적 경사 하강에서, 위의 최소 최대 최적화는 C를 훈련하기 위해 C에 대한 손실 함수의 그라디언트를 취하고 손실 함수의 값이 감소하도록 파라미터를 업데이트한다는 것을 의미한다.그라디언트는 다음과 같다:E[ CC L(x, Mc ) + α C 로그(1 D(C(x, Mc )))]"
"(5)In practice, we take a more (cid:27)ne-grained control, such as initiallykeeping the norm of the MSE loss gradient roughly the same orderof magnitude as the norm of the discriminator gradient. This helpsstabilize the learning"
"(5) 실제로, 우리는 MSE 손실 구배의 규범을 식별자 구배의 규범과 대략 동일한 크기의 순서로 초기에 유지하는 것과 같이 더 많은 (cid : 27)ne-grained 제어를 취한다.이것은 학습을 안정시키는데 도움이 된다"
"We also update the discriminator networks D similarly, except wetake update in the opposite direction so that the loss increases. Notethat here D consists of the local and the global context discriminators"
또한 손실이 증가하도록 반대 방향으로 업데이트하는 것을 제외하고 차별자 네트워크 D를 유사하게 업데이트한다.여기서 D는 로컬 및 글로벌 컨텍스트 판별기로 구성됩니다
"So the (cid:30)ow of the gradient in backpropagation initially splits intothe two networks and then merge into the completion network. In optimization, we use the ADADELTA algorithm [Zeiler 2012],which sets a learning rate for each weight in the network automati-cally"
"그래서 역확산에서의 구배의 (cid:30)ow는 처음에는 두 네트워크로 분할된 다음 완료 네트워크로 병합된다.최적화에서는 ADADELTA 알고리즘[Zeiler 2012]을 사용하는데, 이 알고리즘은 네트워크에서 각 가중치에 대한 학습 속도를 자동으로 설정한다."
Table 4. Analysis of computation time of our model
표 4. 모형의 계산시간 분석
" We notice a significantspeedup when using the GPU that drives computation times down to undera second even for large input images. Image SizePixels CPU (s) GPU (s)Speedup512 × 512768 × 7681024 × 1024409,600589,8241,048,5762.2864"
"우리는 큰 입력 이미지에도 계산 시간을 1초 이하로 낮추는 GPU를 사용할 때 상당한 속도 향상을 알아차린다.이미지 크기 픽셀 CPU(s) GPU(s)Speedup512 512768 7681024 1024409,600589,8241,048,5762.2864"
9338.2620. 1410.3120
9338.2620. 1410.3120
56116.2×15. 8×14.7×not very stable
56116.215. 814.7은 매우 안정적이지 않다.
" Unlike other approaches that focus on image gener-ation [Salimans et al. 2016], our method does not generate imagesfrom noise"
"이미지 생성에 초점을 맞춘 다른 접근법과는 달리 [살리만 외].2016년, 우리의 방법은 잡음으로부터 이미지를 생성하지 않는다."
" That helps the training process to be initially more stable. However, since the image completion task itself is very challenging,much care has to be still taken in order to train the networks toconvergence"
그것은 훈련 과정이 처음에는 더 안정적이 되도록 돕는다.그러나 이미지 완성 작업 자체가 매우 어렵기 때문에 네트워크 수렴을 위해 많은 주의가 필요합니다
"An overview of the general training procedure can be seen inAlgorithm 1. The training is split into three phases: (cid:27)rst, the com-pletion network is trained with the MSE loss from Eq"
"일반 훈련 절차의 개요는 Algorithm 1에서 볼 수 있습니다.훈련은 (cid : 27)rst의 세 단계로 나뉘며, com-pletion 네트워크는 Eq에서 MSE 손실로 훈련됩니다."
" (2) for TCiterations. Afterwards, the completion network is (cid:27)xed and thediscriminators are trained from scratch for TD iterations"
"(2) TCiterations.그 후, 완료 네트워크는 (cid : 27)xed되고 판별자는 TD 반복을 위해 처음부터 훈련됩니다."
" Finally,both the completion network and content discriminators are trainedjointly until the end of training. The pretraining of the completionand the discriminator networks has proved critical for successfultraining"
"마지막으로, 완료 네트워크와 콘텐츠 판별기는 모두 훈련이 끝날 때까지 공동으로 훈련됩니다.완성과 판별 네트워크의 사전 훈련은 성공적인 훈련에 매우 중요하다는 것이 증명되었다."
"In order to facilitate the propagation of gradients through the net-work, during training we use the batch normalization layers [Io(cid:29)eand Szegedy 2015] after all convolutional layers except for the lastlayers of both the completion and the discriminator networks. Thisnormalizes the output of each layer using output statistics that areupdated online"
그물망을 통한 경사도의 전파를 용이하게 하기 위해 훈련 중 완성도와 판별기 네트워크의 마지막 층을 제외한 모든 컨볼루션 층을 제외한 배치 정규화 층[Io(cid:29)eand Szegedy 2015]을 사용한다.이 정규화는 온라인에서 업데이트된 출력 통계를 사용하여 각 계층의 출력을 정규화합니다
" During testing, they can be integrated into the pre-ceding convolutional layer, so as not to add computational burden. Training is done by resizing images so that the smallest edge is arandom value in the [256, 384] pixel range"
"테스트하는 동안, 그들은 계산 부담을 가중시키지 않도록 사전-시딩 컨볼루션 층에 통합될 수 있다.[256, 384] 픽셀 범위 내에서 가장 작은 에지가 랜덤 값이 되도록 이미지를 크기 조정하여 트레이닝을 수행한다"
" Afterwards, a random256 × 256-pixel patch is extracted and used as the input image. Forthe mask, we generate a random hole in the [96, 128] pixel rangeand (cid:27)ll it with the mean pixel value of the training dataset"
"이후 랜덤 256 , 256 픽셀 패치가 추출되어 입력 이미지로 사용됩니다.마스크를 위해, 우리는 [96, 128] 픽셀 범위에 랜덤 홀을 생성하고 (cid:27) 그것을 훈련 데이터 세트의 평균 픽셀 값으로 생성한다."
" Note thatthe aspect ratio of this hole can vary as the width and height aredrawn separately. The input for the global context discriminator isthe full 256 ×256-pixel image, and for the local context discriminatorthe input is a 128 × 128-pixel patch centered around the completedregion (or a random area for real samples)"
"이 구멍의 종횡비는 폭과 높이가 별도로 그려짐에 따라 다를 수 있습니다.글로벌 컨텍스트 판별기의 입력은 전체 256 256 픽셀 이미지이며, 로컬 컨텍스트 판별기의 경우 입력은 완료된 지역(또는 실제 샘플의 랜덤 영역)을 중심으로 한 128 128 픽셀 패치다."
3.5. 1Simple post-processing
1 간단한 후처리
" Although our network model canplausibly (cid:27)ll missing regions, sometimes the generated area hassubtle color inconsistencies with the surrounding regions. To avoidthis, we perform simple post-processing by blending the completedregion with the color of the surrounding pixels"
"네트워크 모델이 가능할 정도로 (cid : 27) l l l loss regions이지만, 생성된 영역은 주변 영역과의 색 불일치가 있는 경우가 있습니다.이를 피하기 위해, 우리는 완성된 영역을 주변 픽셀의 색상과 혼합함으로써 간단한 후처리를 수행한다."
" In particular, weemploy the fast marching method [Telea 2004], followed by Poissonimage blending [Pérez et al. 2003]"
"특히, Weemploy는 빠른 행진 방법[Telea 2004], Poissonimage blending [Pérez et al.2003년]"
"3.5 Stable TrainingDuring the training, the context discriminators are trained to dis-tinguish fake from real images, while the completion network istrained to deceive the discriminators. As the optimization consistsof jointly minimizing and maximizing con(cid:30)icting objectives, it is4 RESULTSWe train our model using 8, 097, 967 training images taken from thePlaces2 dataset [Zhou et al"
"3.5 안정적 훈련훈련과정에서 상황판별자는 실제 이미지에서 가짜를 분리하도록 훈련받고, 완성 네트워크는 차별자를 속이도록 훈련받는다.최적화는 공동으로 콘(cid:30)을 최소화하고 최대화하는 것으로 구성되므로, 4 ReultSULSTSWe는 Places2 데이터셋[Zhou et al]에서 가져온 8, 097, 967 훈련 이미지를 사용하여 모델을 훈련한다."
2016]. This dataset includes images of adiversity of scenes and was originally meant for scene classi(cid:27)cation
2016].이 데이터 세트에는 장면의 다양성 이미지가 포함되어 있으며 원래 장면 클래스 (cid : 27) 양이온을 위한 것이 었습니다.
"We set the weighting hyper-parameter to α = 0.0004, and train usingACM Transactions on Graphics, Vol. 36, No"
"가중 하이퍼 파라미터를 α = 0.0004로 설정하고, 그래픽스, Vol.에 대한 ACM 트랜잭션을 이용하여 열차를 운행한다.36, 아니"
" 4, Article 107. Publication date: July 2017"
제107조 출판일: 2017년 7월
Globally and Locally Consistent Image Completion •107:7tupnIhctaMhctaPgnidleMegamI]4102. lategnauH[]6102
전지구적 및 국지적 일관성 이미지 완성 •107:7tupnIhctaMhctaPgnidleMegamI]4102.
latekahtaP[sruOFig. 4
라테카타프[sruOFig. 4
" Comparisons with existing works. We compare with Photoshop Content Aware Fill (PatchMatch), Image Melding, [Huang et al"
"기존 작품과의 비교.우리는 포토샵 콘텐츠 인식 채우기(패치매치), 이미지 멜딩, [황 등]과 비교한다."
" 2014], and [Pathak et al. 2016] using random masks"
"2014년), [Pathak et al. 2016] 랜덤 마스크 사용"
" For the comparison, we have retrained the model of [Pathak et al. 2016] on the Places2 dataset for arbitrary region completion"
비교를 위해 [Pathak et al.임의의 영역 완료를 위한 Places2 데이터 세트 2016]
"Furthermore, we use the same post-processing as used for our approach. We can see that, while PatchMatch and Image Melding generate locally consistentpatches extracted from other parts of the image, they are not globally consistent with the other parts of the scene"
"또한, 우리는 우리의 접근에 사용된 것과 동일한 후처리를 사용한다.패치매치와 이미지 멜딩이 이미지의 다른 부분에서 추출한 로컬 일관된 패치를 생성하지만, 그것들은 전세계적으로 장면의 다른 부분과 일치하지 않는다는 것을 알 수 있다."
" The approach of [Pathak et al. 2016] caninpaint novel regions, but the inpainted region tends to be easy to identify, even with our post-processing"
"[Pathak et al.2016] 새로운 영역을 페인트칠하지만, 페인트칠된 영역은 사후 처리에도 쉽게 식별할 수 있는 경향이 있다."
" Our approach, designed to be both locally andglobally consistent, results in much more natural scenes. Photographs courtesy of Katja Hasselkus (Public Domain), Mississippi Department of Archives andHistory (Public Domain), Sprachenatelier Berlin (CC0), and Sami Alhammad (Public Domain)"
"지역적으로나 지구적으로 일관되게 설계된 우리의 접근방식은 훨씬 더 자연스러운 장면을 만들어낸다.사진 제공: 카타 하셀쿠스(공공 도메인), 미시시피 기록 및 역사부(공공 도메인), 스프레케나틀리에 베를린(CC0), 사미 알함마드(공공 도메인)"
" Additional results can be found in the supplemental materials. ACM Transactions on Graphics, Vol"
추가적인 결과는 보충재료에서 찾을 수 있다.
" 36, No. 4, Article 107"
"36, 제4조, 제107조"
" Publication date: July 2017. 107:8• Satoshi Iizuka, Edgar Simo-Serra, and Hiroshi Ishikawa(a) Input(b) PM(c) IM(d) SC(e) CE(f) Ours (CM)(g) Ours(h) GTFig"
"출판일 : 2017년 7월.Iizuka 사토시, Edgar Simo-Serra, Hiroshi Ishikawa(a) 입력 (b) PM (c) IM (d) SC (e) CE (f) 우리 (CM) (g) 우리 (h) GTFig"
5. Comparison with the Context Encoder (CE) [Pathak et al
5. 상황 부호화기(CE)[Pathak et al.
2016] on images taken from the ImageNet validation set for center region completion. Allimages are resized to 128 × 128 pixels and the center 64 × 64 pixel region is completed
2016] 중앙 영역 완료를 위한 이미지넷 유효성 검사 세트에서 가져온 이미지에 대한 정보.모든 이미지는 128 픽셀로 크기 조정되고 센터 64 64 픽셀 영역은 완료됩니다
Both CE and Ours (CM) are trained on the same 100k subset of trainingimages of ImageNet to complete the fixed center masks. Ours is our full model trained on the Places2 dataset using higher resolution images for arbitraryregion completion and not only center region completion at a fixed resolution unlike the CE and Ours (CM) models
CE와 Ours(CM)는 고정된 센터 마스크를 완성하기 위해 이미지넷의 동일한 100k 하위 세트의 훈련 이미지에서 훈련된다.우리의 모델은 임의의 영역 완료를 위해 고해상도 이미지를 사용하여 Places2 데이터셋에서 훈련된 전체 모델이며 CE 및 Ours(CM) 모델과 달리 고정 해상도로 중앙 영역 완료뿐만 아니라
" We also provide results for PatchMatch(PM), Image Melding (IM), and [Huang et al. 2014] (SC) for the sake of completion"
"또한 PatchMatch(PM), Image Melding(IM), Huang et al.2014](SC) 완료를 위한"
" The first two rows show examples in which our model trained on ImageNetperforms be(cid:29)er, while the next two rows show examples which obtain roughly the same performance, while the final row shows an example in which CEoutperforms our model. Additional results can be found in the supplemental materials"
"처음 두 행은 이미지넷 수행에서 훈련된 모델이 (cid:29)er인 예를 보여주는 반면, 다음 두 행은 대략 같은 성능을 얻는 예를 보여주는 반면, 마지막 행은 CEoutperforms가 우리의 모델을 수행하는 예를 보여준다.추가적인 결과는 보충 재료에서 찾을 수 있다"
"a batch size of 96 images. The completion network is trained forTC = 90, 000 iterations; then the discriminator is trained for TD =10, 000 iterations; and (cid:27)nally both are jointly trained to reach thetotal of Tt r ain = 500, 000 iterations"
"96개의 이미지 배치 크기입니다.완료 네트워크는 TC = 90,000 반복을 위해 훈련된다. 그런 다음 판별기는 TD = 10,000 반복을 위해 훈련된다. (cid:27) 둘 다 Tt r = 500,000 반복의 총계에 도달하도록 공동으로 훈련된다."
" The entire training proceduretakes roughly 2 months on a single machine equipped with fourK80 GPUs. We evaluate our model using images from a wide variety ofscenes not used in the training data, and compare with the existingapproaches, demonstrating the performance of our method"
전체 훈련 절차는 4K80 GPU가 장착된 단일 기계에서 약 2개월이 걸립니다.훈련 데이터에 사용되지 않는 다양한 장면의 이미지를 사용하여 모델을 평가하고 기존 접근 방식과 비교하여 방법의 성능을 입증합니다
" Unlessotherwise mentioned, our models are trained on the Places2 dataset. 4.0"
"다른 언급이 없다면, 우리의 모델은 Places2 데이터 세트에서 훈련된다. 4.0"
"1 Computational time. Processing time of image completiondepends on the resolution of the input image, not on the size ofthe region to be completed"
1 계산 시간.이미지 완료 처리 시간은 입력 이미지의 해상도에 따라 달라지며 완료할 영역의 크기에 따라 달라지지 않습니다
Table 4 shows the computation timefor several resolutions. We evaluate both on CPU and GPU usingan Intel Core i7-5960X CPU @ 3.00 GHz with 8 cores and NVIDIAGeForce TITAN X GPU
표 4는 여러 해상도에 대한 계산 시간을 보여줍니다.우리는 8개의 코어와 NVIDIAGeForce TITAN X GPU를 가진 Intel Core i7-5960X CPU @ 3.00 GHz를 사용하여 CPU와 GPU에서 모두 평가한다.
" Even large images can be processed inunder a second using a GPU. 4.1 Comparison with Existing WorkWe evaluate our approach on both the general arbitrary regioncompletion, and the center region completion task of [Pathak et al"
심지어 큰 이미지도 GPU를 사용하여 1초 이내에 처리할 수 있다.4.1 기존 작업과의 비교 우리는 일반적인 임의의 영역 완성과 [Pathak et al]의 중심 영역 완성 작업에 대한 우리의 접근법을 평가한다.
2016]. 4.1.1 Arbitrary Region Completion
2016년.
" We compare our results withPhotoshop Content Aware Fill that uses PatchMatch [Barnes et al. 2009], Image Melding [Darabi et al"
"우리는 우리의 결과를 패치매치[Barnes et al.]를 사용하는 포토샵 콘텐츠 인식 필과 비교한다.2009), 이미지 멜딩 [Darabi et al."
" 2012], [Huang et al. 2014], and[Pathak et al"
"2012), [Huang et al. 2014], 및 [Pathak et al."
" 2016]. For the comparison, we have retrained themodel of [Pathak et al"
2016) 비교를 위해 [Pathak et al]의 모델을 재교육했다.
" 2016] on the Places2 dataset for arbitrarymasks for the same number of epochs as our model, and use thebest performing model obtained during training. We evaluate it byresizing the images to its (cid:27)xed input size, processing, resizing backto the original size, and restoring the pixels outside of the mask"
"2016] Places2 데이터 세트에서 임의 마스크에 대해 우리 모델과 동일한 수의 Epoch에 대해, 훈련 중에 얻은 최고의 성능 모델을 사용한다.우리는 이미지를 (cid : 27)xed 입력 크기로 재조정하고, 처리하고, 원래 크기로 재조정하고, 마스크 외부의 픽셀을 복원하여 평가합니다"
"Furthermore, we use the same post-processing as our approach,which is essential for obtaining results. Results are shown in Fig"
"게다가, 우리는 우리의 접근방식과 같은 후처리를 사용하는데, 이것은 결과를 얻기 위해 필수적이다.결과는 그림으로 표시됩니다"
" 4. The patch-based approaches areunable to generate novel objects in the image, unlike our approach"
4.패치 기반 접근 방식은 우리의 접근 방식과 달리 이미지에서 새로운 객체를 생성할 수 없습니다
"ACM Transactions on Graphics, Vol. 36, No"
"그래픽에 대한 ACM 거래, Vol. 36, No"
" 4, Article 107. Publication date: July 2017"
제107조 출판일: 2017년 7월
Globally and Locally Consistent Image Completion •107:9(a) Input(b) Weighted MSE(c) Global D(d) Local D(e) Full methodFig. 6
전역 및 지역 일관된 이미지 완료 •107 : 9 (a) 입력 (b) 가중 MSE (c) Global D (d) Local D (e) Full methodFig.6
" Comparison of training with di(cid:27)erent discriminator configurations. We show the results of models trained with di(cid:27)erent discriminator configurations:(b) Weighted MSE (no discriminators), (c) using Weighted MSE and only a global discriminator, (d) using Weighted MSE and only a local discriminator, and (e)using Weighted MSE and both the global and the local discriminator"
"di(cid:27)erent discriminator 구성과의 훈련 비교.우리는 di(cid:27)erent discriminator 구성으로 훈련된 모델의 결과를 보여준다: (b) 가중 MSE (discriminator)를 사용하지 않고, (c) 가중 MSE와 전역 discriminator만을 사용하고, (d) 가중 MSE와 국부 discriminator만을 사용하고, (e) 가중 MSE와 전역 및 국부 discriminator를 모두 사용하여"
" Photographs courtesy of rente42 (Public Domain), and Pete (Public Domain). Furthermore, while they are able to complete with locally consistentimage patches, they are not necessarily globally consistent with thescene, e"
"사진은 렌터카42(공공 도메인), 피트(공공 도메인)의 제공.또한, 로컬 일관된 이미지 패치로 완료할 수 있지만, 반드시 전 세계적으로 이 장면과 일치하는 것은 아닙니다."
"g. , objects may appear in mid-air or in the middle of otherobjects"
g. 물체는 공중 또는 다른 물체의 중간에 나타날 수 있습니다.
" The model of [Pathak et al. 2016] results in blurred and easyto identify areas, even with our post-processing"
[Pathak et al.2016년)은 사후 처리에도 불구하고 영역을 식별하기 쉽고 흐릿하게 만든다.
" Our approach isexplicitly trained to be both locally and globally consistent, leadingto much more natural image completion. 4.1.2 Center Region Completion"
우리의 접근방식은 지역적으로나 세계적으로 일관성을 갖도록 훈련되어 훨씬 더 자연스러운 이미지 완성을 이끌어낸다.4.1.2 센터 지역 완료
" We also compare with the Con-text Encoder (CE) [Pathak et al. 2016] on their provided 128 × 128-pixel test images, taken from ImageNet [Deng et al"
또한 Con-text Encoder (CE) [Pathak et al.2016] ImageNet [Deng et al]에서 가져온 128 픽셀 테스트 이미지 제공
" 2009], with the(cid:27)xed 64 × 64-pixel inpainting masks in the center of the image. For afair comparison, we train our model using their training data, whichconsists of a subset of 100K images of ImageNet, for 500 epochs"
"2009년, (cid : 27)xed 64 64 픽셀 인페인팅 마스크가 이미지 중앙에 있습니다.공정한 비교를 위해, 우리는 이미지넷의 100K 이미지의 하위 집합으로 구성된 그들의 훈련 데이터를 사용하여 500개의 시대를 위해 우리의 모델을 훈련시킨다."
Wealso do not perform post-processing for the results of our model. Results are shown in Fig
또한 모형의 결과에 대한 후처리를 수행하지 않습니다. 결과는 그림에 나와 있습니다
" 5. For the center region completion task,the results of CE are signi(cid:27)cantly better than in the general arbi-trary region completion case"
"5.중심 영역 완성 작업의 경우, CE의 결과는 일반 아르비-트레이 영역 완성 사례보다 훨씬 더 좋다."
" We provide many more results in thesupplemental material and encourage the reader to look at them toappreciate the relative performance of our technique and of CE. Wenote that, while the CE approach is specialized to inpaint images ofthis size and (cid:27)xed holes, our model is capable of arbitrary regioncompletion at any resolution"
"우리는 보충 자료에 더 많은 결과를 제공하고 독자가 우리의 기술과 CE의 상대적 성능을 평가하기 위해 그들을 보도록 권장합니다.CE 접근법은 이 크기와 (cid : 27)xed 구멍의 이미지를 페인트하는 데 전문화되어 있지만, 우리 모델은 임의의 해상도에서 임의의 영역 완료를할 수 있습니다."
" We also show results of our full modeltrained on the Places2 dataset using higher resolution images forarbitrary region completion. We note that the other two models aretrained exclusively for this particular task of center region comple-tion at the (cid:27)xed resolution of 128 × 128 pixels, while this model cancomplete arbitrary regions of images of any resolution"
"또한, 우리는 임의 영역 완료를 위해 고해상도 이미지를 사용하여 Places2 데이터 세트에서 전체 모델의 결과를 보여준다.우리는 다른 두 모델이 128 x 128 픽셀의 (cid : 27)x 해상도에서 중심 영역 보완의 특정 작업을 위해 독점적으로 훈련되는 반면이 모델은 해상도의 임의의 이미지 영역을 완성할 수 있다는 점에 주목합니다."
" For the sakeof completion, the results of patch-based approaches [Barnes et al. 2009; Darabi et al"
이를 위해 패치 기반 접근법을 적용하였다 [Barnes et al. 2009; Darabi et al.
2012; Huang et al. 2014] are also provided in thecomparison
2012; Huang et al. 2014]은 비교에서도 제공됩니다.
4.2 Global and Local ConsistencyWe investigate the in(cid:30)uence of the global and the local contextdiscriminators by training models that only use one of them andcomparing with the full approach. We show the results in Fig
4.2 글로벌 및 로컬 일관성 우리는 그 중 하나만 사용하는 모델을 훈련하고 전체 접근법과 비교함으로써 글로벌 및 로컬 컨텍스트 판별 자의 in (cid : 30uence)을 조사합니다.우리는 그 결과를 그림으로 보여줍니다
" 6. We can see that, when the local discriminator is not used (b)(c),the result is completion by large blurred areas"
6.지역 판별기를 사용하지 않을 경우 (b)(c)를 사용하여 결과가 흐릿한 영역으로 완성됨을 알 수 있다.
" On the other hand,(a) Input(b) Output(c) Post-processedFig. 7"
반면에 (a) 입력(b) 출력(c) 후처리된 그림.
" E(cid:27)ect of our simple post-processing. while using only the local discriminator (d) results in locally morerealistic textures, without the global discriminator it still lacks globalconsistency"
"E (cid : 27) 우리의 간단한 후 처리.지역 판별기(d)만 사용하면 지역적으로 더 현실적인 질감이 발생하지만, 글로벌 판별기가 없으면 여전히 글로벌 일관성이 부족하다."
" By using both the global and the local discriminators,we can achieve results that are both locally and globally consistent. 4.3 E(cid:27)ect of Post-Processing and Training DataWe show the e(cid:29)ect of our simple post-processing in Fig"
글로벌 차별자와 로컬 차별자를 모두 사용함으로써 우리는 지역적으로나 세계적으로 일관된 결과를 얻을 수 있습니다.4.3 사후 처리 및 훈련 데이터의 E (cid : 27) ect 우리는 그림에서 간단한 후 처리의 e (cid : 29) ect를 보여줍니다.
7. Wecan see how this simple post-processing can be used to make theinpainted area blend better into the global image
7.우리는 이 간단한 후처리가 어떻게 페인트칠된 영역을 글로벌 이미지와 더 잘 혼합시키는 데 사용될 수 있는지 알 수 있다.
"We also look at the e(cid:29)ect of the dataset used for training ourmodel. In particular, we compare models trained on Places2 [Zhouet al"
또한 모델 교육에 사용되는 데이터 집합의 e(cid:29) ect를 살펴 봅니다.특히 Places2 [Zhouet al]에서 훈련된 모델을 비교한다.
2016] and ImageNet [Deng et al. 2009]
2016] 및 ImageNet [Deng et al. 2009]
" The Places2 datasetconsists of roughly 8 million images of scenes, while the ImageNetdataset focuses on classi(cid:27)cation on objects and only has 1 millionimages. Results are shown in Fig"
"Places2 데이터셋은 약 800만 개의 장면 이미지를 구성하며, ImageNetdataset은 객체에 대한 클래스(cid:27) 양이온에 초점을 맞추고 있으며 100만 개의 이미지만 가지고 있다.결과는 그림으로 표시됩니다"
" 8. Although results are fairlysimilar, the results of the model trained on Places2 gives betterperformance in a wide diversity of scenarios, and is the primarymodel we use unless stated otherwise"
"8.결과는 상당히 유사하지만, Places2에서 훈련된 모델의 결과는 다양한 시나리오에서 더 나은 성능을 제공하며, 달리 명시하지 않는 한 우리가 사용하는 기본 모델이다."
4.4 Object RemovalOne of the main motivations of image completion is being able toremove unwanted objects in images. We show examples of objectremoval in Fig
4.4 이미지 완성의 주요 동기 중 하나는 이미지에서 원하지 않는 객체를 제거할 수 있다는 것이다.우리는 그림에서 물체 제거의 예를 보여준다.
" 9. The results of our approach are natural and itACM Transactions on Graphics, Vol"
"본 연구의 결과는 자연스럽고, 그래픽에 대한 ITACM 거래, Vol."
" 36, No. 4, Article 107"
"36, 제4조, 제107조"
" Publication date: July 2017. 107:10• Satoshi Iizuka, Edgar Simo-Serra, and Hiroshi Ishikawa(a) Input(b) Ours (ImageNet)(c) Ours (Places2)Fig"
"출판일 : 2017년 7월.Iizuka 사토시, Simo-Serra, Ishikawa 히로시 (a) 입력 (b) 우리 (ImageNet) (c) 우리 (Places2) 그림"
8. Results of training with di(cid:27)erent datasets
8. 디(산:27)에렌트 데이터셋을 이용한 훈련 결과
" In particular, we com-pare a model trained on the ImageNet dataset with one trained on thePlaces2 dataset. Photographs courtesy of Bernard Spragg"
"특히, 우리는 이미지넷 데이터셋에서 훈련된 모델을 Places2 데이터셋에서 훈련된 모델로 준비한다.사진 제공 버나드 스프래그"
" NZ (CC0), andBig Cypress National Preserve (Public Domain). (a) Original(b) Input(c) OutputFig"
(a) 원작(b) 입력(c) 출력피그
9. Examples of object removal by our approach
9. 접근방식에 의한 물체제거의 예
" Photographs courtesyof Ginés González de la Bandera (Public Domain), and Katja Hasselkus(Public Domain). becomes nearly impossible to identify where an object has beenremoved"
"사진은 Ginés González de la Bandera(공공 도메인), Katja Hasselkus(공공 도메인)의 제공.물체가 제거된 위치를 식별하는 것이 거의 불가능해짐"
"4.5 Faces and FacadesAlthough our model can generate various texture or objects tocomplete missing regions in general images, (cid:27)ne-tuning the modelusing a speci(cid:27)c dataset can achieve even better results for moreconcrete and complicated image completion tasks. In particular, weconsider both the CelebFaces Attributes Dataset (CelebA) [Liu et al"
"4.5 얼굴과 얼굴 비록 우리의 모델이 일반적인 이미지에서 다양한 질감이나 물체를 생성할 수 있지만, (cid:27) speci(cid:27)c 데이터셋을 사용하여 모델을 조정하면 더 구체적이고 복잡한 이미지 완성 작업에 훨씬 더 나은 결과를 얻을 수 있다.특히, Weconsider both the CelebFaces Attribts Dataset (CelebA) [Liu et al."
"2015], and the CMP Facade dataset [Radim Tyleček 2013], whichconsist of 202, 599 and 606 images, respectively. For both datasets,we use the image completion network trained on the Places2 datasetand further train it on the new data"
"2015), 202, 599 및 606 이미지로 구성된 CMP 파사드 데이터 세트[Radim Tyleek 2013].두 데이터 세트 모두 Places2 데이터 세트에서 훈련된 이미지 완료 네트워크를 사용하고 새 데이터에서 추가 교육"
" To adapt to new data, we initiallytrain the context discriminator from scratch, then both the contextdiscriminator and the completion network are trained together. For the CelebA dataset, we train using 200, 000 images"
"새로운 데이터에 적응하기 위해, 우리는 처음에 컨텍스트 판별기를 처음부터 훈련시킨 다음, 컨텍스트 판별기와 완료 네트워크 둘 다 함께 훈련된다.셀레바 데이터 세트를 위해 우리는 200,000개의 이미지를 사용하여 훈련한다."
" As thedataset has images of 178 × 218 pixels, we slightly adapt the trainingapproach: instead of using 256×256-pixel image patches for training,we use 160 × 160-pixel image patches. We randomly generate holesin the [48, 96]-pixel range and thus modify the input of the localdiscriminator to be 96 × 96 pixels instead of 128 × 128 pixels"
"데이터 세트에는 178개의 218 픽셀 이미지가 있으므로 교육용으로 256256 픽셀 이미지 패치를 사용하는 대신 160개의 160 픽셀 이미지 패치를 사용합니다.우리는 무작위로 [48, 96] 픽셀 범위에 구멍을 생성하여 128 픽셀 대신 96 픽셀로 국소 판별기의 입력을 수정합니다."
" Finally,ACM Transactions on Graphics, Vol. 36, No"
"마지막으로, 그래픽에 대한 ACM 트랜잭션 36, No."
" 4, Article 107. Publication date: July 2017"
제107조 출판일: 2017년 7월
"we remove a layer from the global context discriminator and adaptthe fully-connected layers of both the global and the local contextdiscriminators to the new training resolutions. For the CMP Facade dataset, we train using 550 images"
글로벌 컨텍스트 판별기에서 레이어를 제거하고 글로벌 컨텍스트 판별기와 로컬 컨텍스트 판별기의 완전히 연결된 레이어를 새로운 교육 해상도에 적용합니다.CMP 페이케이드 데이터 세트의 경우 550개의 이미지를 사용하여 훈련합니다
" Thetraining procedure is the same as for the Places2 dataset, except thatthe completion network is initialized with the one trained on thePlaces2 dataset, instead of being trained with the MSE loss for TCiterations. We show the results in Fig"
"훈련 절차는 Places2 데이터 세트와 동일하지만, 완료 네트워크는 TCiterations에 대한 MSE 손실로 훈련되는 대신 Place2 데이터 세트에서 훈련된 것으로 초기화된다.우리는 그 결과를 그림으로 보여줍니다"
10. We can see that our approachcan realistically complete faces despite very large occluded areas
10. 우리는 우리의 접근방식이 매우 넓은 폐쇄된 지역에도 불구하고 현실적으로 완전한 얼굴을 할 수 있다는 것을 알 수 있다.
"Note that patch-based approaches are unable to complete faces, as itrequires the algorithm to generate novel objects such as eyes, noses,and mouths that are not already part of the image. We also see thatour approach can complete various types of facades in a way thatthey are both locally and globally coherent"
"패치 기반 접근법은 이미 이미지의 일부가 아닌 눈, 코 및 입과 같은 새로운 객체를 생성하는 알고리즘을 요구하기 때문에 얼굴을 완성할 수 없습니다.또한 우리는 우리의 접근방식이 지역적으로나 세계적으로 일관성이 있는 방식으로 다양한 형태의 면을 완성할 수 있다는 것을 알 수 있다."
4.6 User StudyWe perform a user study using the validation set of the CelebAdataset for the challenging face completion task and show results inFig. 11
4.6 사용자 연구 도전적인 얼굴 완성 작업에 대한 CelebAdataset의 유효성 확인 세트를 사용하여 사용자 연구를 수행하고 결과를 그림으로 표시합니다.11
" We ask 10 users to evaluate the naturalness of the completion. The users are only shown either the full completed image or arandom image from the dataset, and asked to guess if the image isan actual image from the dataset or a completed one"
우리는 10명의 사용자에게 완성의 자연성을 평가해 줄 것을 요청한다.사용자는 데이터 세트에서 완전 완료된 이미지 또는 임의 이미지만 표시되며 이미지가 데이터 세트에서 실제 이미지인지 또는 완료된 이미지인지 추측하도록 요청합니다
" The (cid:27)gureshows the percentage of the images that are deemed to be real. Thatis, 77.0% of the completed images by our approach is thought tobe real"
"(cid : 27) gures는 실제로 간주되는 이미지의 비율을 보여줍니다.즉, 우리의 접근방식에 의해 완성된 이미지의 77.0%가 실제라고 생각된다."
" For comparison, the real images are correctly categorized96.5% of the time. This highlights the realism of the resulting imagecompletion by our approach"
비교를 위해 실제 이미지는 시간의 96.5%로 정확하게 분류된다.이것은 우리의 접근방식에 의한 결과적인 이미지 완성의 사실성을 강조한다.
4.7 Additional ResultsWe show additional results for our approach in Fig. 12
4.7 추가 결과 그림에서 접근 방법에 대한 추가 결과를 보여줍니다.
" Our ap-proach can complete a wide diversity of scenes such as mountainranges, close ups of walls, and churches. Furthermore, the resultslook natural even when large sections of the image are completed"
"우리의 ap-proach는 산악지대, 벽의 클로즈업, 교회와 같은 다양한 장면들을 완성할 수 있다.또한, 이미지의 큰 부분이 완성된 경우에도 결과는 자연스러워 보입니다"
"4.8 Limitations and DiscussionAlthough our model can handle various images of any sizes witharbitrary holes, signi(cid:27)cantly large holes cannot be (cid:27)lled in due to thespatial support of the model as discussed in Section 3. 2"
"4.8 한계와 논의 비록 우리의 모델이 임의의 구멍이 있는 크기의 다양한 이미지를 처리할 수 있지만, 3절에서 논의한 모델의 공간적 지원으로 인해 큰 구멍은 (cid:27) lled 될 수 없다.2"
" By changingthe model architecture to include more dilated convolutions it ispossible to push this limit. Note that this limitation refers strictly tosquare masks, e"
모델 아키텍처를 확장된 컨볼루션을 포함하도록 변경함으로써이 한계를 푸시하는 것이 가능합니다.이 제한은 엄밀히 말하면 제곱 마스크를 가리킨다.
"g. , wide areas can still be completed as long as theyare not too tall: information from above and below will be used tocomplete the image"
"G., 넓은 영역은 너무 높지 않은한 여전히 완료 될 수 있습니다. 위와 아래의 정보는 이미지를 완성하는 데 사용됩니다."
" This is especially limiting in the case of imageextrapolation, in which the inpainting mask is at the border of theimage. Figure 13-left shows such an example, which is from the[Hays and Efros 2007] dataset"
이것은 특히 인페인팅 마스크가 이미지의 경계에 있는 이미지 외삽의 경우 제한적입니다.그림 13-왼쪽은 [Hays and Efros 2007] 데이터 세트에서 나온 그러한 예를 보여줍니다.
" Not only is the missing area verylarge relative to the image, but information from only one side ofthe area is available. Figure 13-right shows another failure case dueto a large inpainting region"
"누락된 영역은 이미지에 비해 매우 클 뿐만 아니라, 그 영역의 한쪽에서만 정보를 이용할 수 있다.그림 13 오른쪽은 페인트칠 영역이 넓어 다른 실패 사례를 보여줍니다"
" We note that in this case, [Hays andEfros 2007] also fails to realistically inpaint the mask. Approacheslike [Hays and Efros 2007], which leverage sizable databases tocopy and paste large parts of images, work well if the databasecontains an image similar to the input"
우리는 이 경우 [Hays and Efros 2007]도 마스크를 현실적으로 칠하지 못한다는 점에 주목한다.상당한 크기의 데이터베이스 토코피를 활용하여 이미지의 많은 부분을 붙여주는 [Hays and Efros 2007]과 같은 접근 방식은 데이터베이스가 입력과 유사한 이미지를 포함하고 있는 경우 잘 작동한다.
" Indeed, for such approaches,extrapolation is easier than inpainting, since there are less to matchGlobally and Locally Consistent Image Completion •107:11Fig. 10"
"실제로 이러한 접근 방식의 경우, 색인보다 색인화가 더 쉽습니다. 왜냐하면 지구 및 지역 일관된 이미지 완성 • 107 : 11Fig에 필적할 수 있기 때문입니다.10"
Faces and Facades. We also apply our model to more specific datasets such as human faces and building facades by fine-tuning on di(cid:27)erent datasets
"얼굴과 얼굴.또한 우리는 우리의 모델을 인간의 얼굴과 같은 보다 구체적인 데이터 세트에 적용하고, di(cid:27)에 대한 미세 조정을 통해 외관을 구축한다."
"In the first two rows we show results of a model trained on the CelebA dataset, while the last row shows results of a model trained on the CMP Facadedataset. The inpainting masks are randomly chosen"
"처음 두 행에서 우리는 CelebA 데이터 세트에서 훈련된 모델의 결과를 보여주는 반면, 마지막 행은 CMP Facadedataset에서 훈련된 모델의 결과를 보여준다.그 도장 마스크들은 무작위로 선택된다"
" Photographs courtesy of SKV Florbal (Public Domain), U. S"
"사진 제공 SKV Florbal(공공 도메인), U.S."
" Department of Agriculture (Public Domain),SKV Florbal (Public Domain), Mo Che (Public Domain), Embajada de los Estados Unidos en Uruguay (Public Domain), Efd Initiative (CC0), Fiona White(Public Domain), Paradox Wolf (Public Domain), thinkrorbot (Public Domain), and Darrell Neufeld (Public Domain). Additional results can be found in thesupplemental materials"
"농림부(공공도메인), SKV 플로발(공공도메인), 모체(공공도메인), 엠바자다 데 로스 에스타도스 유니도스(공공도메인), 에프드 이니셔티브(CC0), 피오나 화이트(공공도메인), 파라독스 울프(공공도메인), 사상로봇(공공도메인), 그리고 대럴 뉴펠드(공공 도메인).추가 결과는 보충 재료에서 찾을 수 있습니다"
Method Naturalness(median)96.5%77.0%GTOursFig. 11
방법 자연성(median)96.5%77.0%GTOursFig.
Result of our user study evaluating the naturalness of the imagecompletion on the CelebA dataset. The numbers are the percentage of theimages that are deemed to be real by 10 di(cid:27)erent users for the Ground Truth(GT) and the result of the completion by our approach
사용자 연구 결과는 CelebA 데이터 세트에서 이미지 완성의 자연성을 평가합니다.수치는 지상진실(GT)에 대한 10개의 di(cid:27)erent 사용자에 의해 실제로 간주되는 이미지의 비율과 우리의 접근에 의한 완료 결과물이다.
"at the boundary. Note that, in the output by [Hays and Efros 2007],parts of the original image outside of the mask are modi(cid:27)ed by(cid:27)tting the image patch from the database"
경계에서.[Hays and Efros 2007]에의 한 출력에서 마스크 외부의 원래 이미지 부분은 데이터베이스에서 이미지 패치를 (cid : 27)팅하여 modi (cid : 27)로 수정됩니다.
"We conducted a user study on this dataset, using its standardprotocol, that compares our approach, Context Encoder (CE) [Pathaket al. 2016], and [Hays and Efros 2007]"
"본 논문에서는 표준 프로토콜을 사용하여 본 데이터 세트에 대한 사용자 연구를 수행하여, 우리의 접근 방식인 Context Encoder (CE) [Pathaket al.2016년, [Hays and Efros 2007]"
" For CE, we use the modelwe retrained for arbitrary masks on the Places2 dataset, as well asour post-processing. A total of 11 users were asked to classify theimages as real or manipulated"
"CE의 경우, 우리는 Places2 데이터 세트의 임의 마스크와 사후 처리를 위해 재교육된 모델을 사용한다.총 11명의 사용자들에게 이미지를 실제 또는 조작된 것으로 분류하도록 요청하였다."
The time taken to discern whetheror not an image is manipulated is shown in Fig. 14
이미지가 조작되지 않는지 여부를 식별하는 데 걸리는 시간을 그림 14에 표시한다.
" As many imageshave large holes at the edge of the map, we also evaluate on a subsetof 19 images in which the holes are centered and not on the edgeof the image. With large holes at the edge of the image, [Hays andEfros 2007] shows much better performance than when they arecentered on the image, and the high resolution of this dataset provea challenge for our approach"
"많은 이미지가지도의 가장자리에 큰 구멍을 가지고 있기 때문에, 우리는 구멍이 이미지의 가장자리가 아닌 중심이 되는 19개의 이미지의 하위 집합에서도 평가합니다.이미지 가장자리에 큰 구멍이 있는 [Hays and Efros 2007]은 이미지에 집중할 때보다 훨씬 더 나은 성능을 보여 주며이 데이터 세트의 높은 해상도는 우리의 접근에 대한 도전을 증명합니다."
" Of the neural network approaches,our approach is harder to distinguish as fake than that of CE, whichsurprisingly performs worse for the interpolation subset of images. The main advantage of our approach over standard techniquessuch as PatchMatch lies in the fact that our approach can generatenovel objects that do not appear in the image"
"신경망 접근법 중, 우리의 접근법은 CE보다 가짜로 구별하기가 더 어려워서, 놀랍게도 이미지의 보간 부분집합에 대해 더 나쁜 성능을 발휘한다.패치매치와 같은 표준 기법보다 우리의 접근방식의 주된 장점은 우리의 접근방식이 이미지에 나타나지 않는 새로운 물체를 생성할 수 있다는 사실에 있다."
" While this may not benecessary for certain outdoor scenes when parts of the image canbe used for image completion, for other cases such as completingfaces, it becomes critical, as without being able to generate noses,eyes, mouths, etc. , the completion will fail as shown in Fig"
"이미지의 일부가 이미지 완성에 사용될 수 있는 특정 야외 장면에는 필요하지 않을 수 있지만 얼굴 완성과 같은 다른 경우에는 코, 눈, 입 등을 생성할 수 없는 것처럼 중요해진다., 완료가 그림에서 보듯이 실패합니다"
15. Some examples of failure cases can be seen in Fig
실패 사례의 예로는 그림에서 볼 수 있다.
" 16. In gen-eral, the most common failure case is when a heavily structuredobject, e"
16. 성기(gen-eral)에서 가장 흔한 실패 사례는 구조가 심한 물체인 e
"g. , a person or an animal, is partially masked"
사람 또는 동물인 g.는 부분적으로 가면을 쓴다
" In the leftimage, we can see that the model prioritizes reconstructing the treesin the background over the head of the boy. In the right image,our approach fails to complete the dog"
"왼쪽 이미지에서, 우리는 그 모델이 소년의 머리보다 배경에 있는 나무를 재건하는 것을 우선시한다는 것을 알 수 있다.올바른 이미지에서, 우리의 접근방식은 개를 완성하지 못한다."
" We do not, however, thatstructured textures do get completed as shown in Fig. 12.5 CONCLUSIONWe have presented a novel approach for image completion that pro-duces locally and globally consistent image completions based onconvolutional neural networks"
그러나 우리는 구조화된 질감이 그림에서 보듯이 완성되지 않는다.12.5 결론 우리는 컨볼루션 신경망을 기반으로 로컬 및 글로벌 일관된 이미지 완성을 유도하는 이미지 완성을 위한 새로운 접근법을 제시했습니다.
" We have shown that, by using globaland local context discriminators, it is possible to train models to pro-duce realistic image completion. Unlike the patch-based approaches,ACM Transactions on Graphics, Vol"
"우리는 글로벌 및 로컬 컨텍스트 판별기를 사용하여 현실적인 이미지 완성을 유도하기 위한 모델을 훈련할 수 있음을 보여 주었습니다.패치 기반 접근법과 달리 그래픽에 대한 ACM 트랜잭션, Vol"
" 36, No. 4, Article 107"
"36, 제4조, 제107조"
Publication date: July 2017. OursGT0.00.20
"발행일 : 2017년 7월, OursGT0.00.20"
"40.60. 81,0Naturalness107:12• Satoshi Iizuka, Edgar Simo-Serra, and Hiroshi IshikawaFig"
"40.60. 81,0자연도 107:12 • 이즈카 사토시, 에드가 시모 세라, 이시카와 히로시"
12. Additional image completion results by our approach on image using randomly generated masks
무작위로 생성된 마스크를 이용한 이미지 접근에 의한 추가적인 이미지 완성 결과
" Photographs courtesy of Alex Liivet (CC0), NewcastleLibraries (Public Domain), Yellowstone National Park (Public Domain), Shenandoah National Park (Public Domain), Shenandoah National Park (PublicDomain), thinkrorbot (Public Domain), Alan Levine (CC0), Bruce Caron (Public Domain), Mr. Delirium (Public Domain), Bernard Spragg"
"사진 제공은 알렉스 리벳(CC0), 뉴캐슬 도서관(공공 도메인), 옐로스톤 국립공원(공공 도메인), 셰난도아 국립공원(공공 도메인), 셰난도아 국립공원(공공 도메인), 싱크로봇(공공 도메인), 앨런 레빈(CC0), 브루스 카론(공공 도메인), 델리륨(공공 도메인), 버나드 스프래그"
" NZ (CC0), Gu YanTemple (CC0), Allie G (Public Domain), and gnuckx (CC0). More results can be found in the supplemental materials"
"NZ(CC0), Gu YanTemple(CC0), Allie G(공공 도메인), gnuckx(CC0).추가적인 자료에서 더 많은 결과를 찾을 수 있다"
"ACM Transactions on Graphics, Vol. 36, No"
"그래픽에 대한 ACM 거래, Vol. 36, No"
" 4, Article 107. Publication date: July 2017"
제107조 출판일: 2017년 7월
tupnI]7002sorfEdnasyaH[]6102. latekahtaP[sruOFig
tupnI]7002sorfEdnasyaH[]6102. 라테카타P[sruOFig
13. Failure cases from the dataset of [Hays and Efros 2007]
[Hays and Efros 2007]의 데이터 세트로부터의 실패 사례
" For thecomparison, we have retrained the model of [Pathak et al. 2016] on thePlaces2 dataset for arbitrary regions"
비교를 위해 [Pathak et al.2016] 임의 영역의 Place2 데이터 세트
" The image on the le(cid:28) corresponds toa case of image extrapolation, i. e"
"l(cid:28) 상의 이미지는 이미지 외삽의 경우, i. e."
", the inpainting mask lies on the boundaryof the image. Out of the 51 images in this dataset, 32 have masks thatcorrespond to image extrapolation"
", 인페인팅 마스크는 이미지의 경계에 놓여 있다.이 데이터 세트의 51개 이미지 중 32개 이미지 외삽에 대응하는 마스크가 있습니다"
Additional results can be found in thesupplemental materials. GT (full)GT (19)Hays (full)Hays (19)CE (full)CE (19)Ours (full)Ours (19)Fig
추가 결과는 보충 재료에서 찾을 수 있습니다.GT (풀)GT (19)Hays (풀)Hays (19)CE (풀)CE (19)우리 (풀)우리 (19)피그
14. User study on the [Hays and Efros 2007] dataset
[Hays and Efros 2007] 데이터셋에 대한 사용자 연구
" We compareGround Truth (GT) images, [Hays and Efros 2007] (Hays), CE [Pathak et al. 2016], and our approach"
"우리는 GT(Ground Truth) 이미지, [Hays and Efros 2007] (Hays), CE [Pathak et al.2016), 그리고 우리의 접근"
Users are asked to distinguish whether or not animage has been manipulated. We plot the percentage properly classified asfunction of the maximum response time
사용자들은 이미지가 조작되었는지 여부를 구별하도록 요청 받는다.최대 응답 시간의 함수로 분류된 비율을 올바르게 표시합니다
" The solid line corresponds to thefull dataset, while the do(cid:29)ed line corresponds to a subset of 19 images inwhich the inpainting masks are not at the edges. Lower is be(cid:29)er"
"솔리드 라인은 전체 데이터 세트에 해당하며, do(cid:29) 라인은 인페인팅 마스크가 에지에 있지 않은 19개의 이미지의 서브셋에 해당한다.하측이(cid:29)er이다"
Globally and Locally Consistent Image Completion •107:13tupnI)a(MP)b(MI)c(sruO)d()tf(sruO)e(Fig. 15
"전지구적, 국지적으로 일관된 이미지 완성 •107:13tupnI(MP)b(MI)c(sruO)d()tf(sruO)e(그림).15"
" Comparison with the PatchMatch (PM) and Image Melding (IM). We provide results for our general model (Ours), and our model fine-tunedfor faces (Ours ((cid:28)))"
패치매치(PM) 및 이미지 멜딩(IM)과의 비교.우리는 일반 모델 (우리)과 얼굴 모델에 대한 미세 조정 결과를 제공합니다 (우리 (산 : 28))).
" Patch-based approaches are unable to generate novelobjects in the scene leading to unnatural results. Photographs courtesy ofOwen Lucas (Public Domain), Mon Mer (Public Domain), and SKV Flor-bal (Public Domain)"
"패치 기반 접근방식은 부자연스러운 결과를 초래하는 장면에서 새로운 객체를 생성할 수 없다.사진 제공 오웬 루카스(공공 도메인), 몬 머(공공 도메인), SKV 플로발(공공 도메인)"
Additional results can be found in the supplementalmaterials. our approach can generate novel objects that do not appear else-where in the image
추가 결과는 보충 재료에서 찾을 수 있습니다.우리의 접근방식은 이미지의 다른 곳에 나타나지 않는 새로운 물체를 생성할 수 있다.
" We have provided in-depth comparisons withexisting approaches and show realistic image completion for a largevariety of scenes. Furthermore, we also use our approach to com-plete images of faces and show in a user study that our generatedfaces are indistinguishable from real faces 77% of the time"
"우리는 기존의 접근방식과 심층적인 비교를 제공했고, 다양한 장면에 대한 현실적인 이미지 완성을 보여주었다.또한, 우리는 얼굴의 이미지를 com-plete하고 사용자 연구에서 생성된 얼굴과 실제 얼굴 77%를 구별할 수 없다는 것을 보여주기 위해 우리의 접근법을 사용한다."
"REFERENCESColoma Ballester, Marcelo Bertalmío, Vicent Caselles, Guillermo Sapiro, and JoanVerdera. 2001"
"레퍼런스콜로마 발레스터, 마르셀로 베르탈미오, 비센트 카셀레스, 기예르모 사피로, 조안베데라.2001"
" Filling-in by joint interpolation of vector (cid:27)elds and gray levels. IEEETransactions on Image Processing 10, 8 (2001), 1200–1211"
"벡터 (cid : 27)elds 및 회색 수준의 공동 보간을 통한 채우기.IEEETransactions on Image Processing 10, 8 (2001), 1200–1211"
"Connelly Barnes, Eli Shechtman, Adam Finkelstein, and Dan B Goldman. 2009"
"코넬리 반즈, 일라이 셰히트먼, 아담 핀켈스타인, 댄 B 골드만. 2009년"
" Patch-Match: A Randomized Correspondence Algorithm for Structural Image Editing. ACM Transactions on Graphics (Proceedings of SIGGRAPH) 28, 3 (2009), 24:1–24:11"
"패치 매치 : 구조 이미지 편집을 위한 무작위 대응 알고리즘.그래픽에 대한 ACM 트랜잭션 (SIGGRAPH의 절차) 28, 3 (2009), 24 : 1-24 : 11"
"ACM Transactions on Graphics, Vol. 36, No"
"그래픽에 대한 ACM 거래, Vol. 36, No"
" 4, Article 107. Publication date: July 2017"
제107조 출판일: 2017년 7월
"107:14• Satoshi Iizuka, Edgar Simo-Serra, and Hiroshi IshikawaInputOursGTInputOursGTFig. 16"
"107:14 • 이즈카 사토시, 시모 세라, 이시카와 이시카와 인푸트오어스GTInputOursGTFig."
" Failure cases for our approach where our model is unable to complete heavily structured objects such as people and animals. Photographs courtesy ofPete (Public Domain), and brad pierce (Public Domain)"
"우리의 모델이 사람과 동물과 같은 심하게 구조화된 물체를 완성할 수 없는 우리의 접근방식에 대한 실패 사례.사진 제공 Pete(공공 도메인), Brad Pearce(공공 도메인)"
"Connelly Barnes, Eli Shechtman, Dan B. Goldman, and Adam Finkelstein"
"코넬리 반즈, 일라이 셰히트먼, 댄 B. 골드만, 아담 핀켈슈타인"
2010. TheGeneralized Patchmatch Correspondence Algorithm
일반화된 패치매치 대응 알고리즘
In European Conference onComputer Vision. 29–43
유럽 컴퓨터 비전 회의.
"Marcelo Bertalmio, Guillermo Sapiro, Vincent Caselles, and Coloma Ballester. 2000"
"마르셀로 베르탈미오, 기예르모 사피로, 빈센트 카셀레스, 콜로마 발레스터. 2000"
Image Inpainting. In ACM Transactions on Graphics (Proceedings of SIGGRAPH)
이미지 인페인팅. 그래픽에 대한 ACM 트랜잭션(SIGGRAPH의 절차)
417–424. M
417-424 M
" Bertalmio, L. Vese, G"
"베르탈미오, L. 베세, G"
" Sapiro, and S. Osher"
사피로와 S. 오셔
2003. Simultaneous structure and textureimage inpainting
"2003년, 동시구조와 질감이미지 인페인팅"
" IEEE Transactions on Image Processing 12, 8 (2003), 882–889. A"
"IEEE 거래에 관한 이미지 처리 12, 8(2003), 882-889."
" Criminisi, P. Perez, and K"
"크리미니시, P. 페레즈, 그리고 K"
Toyama. 2004
2004년 도야마
" Region Filling and Object Removal byIEEE Transactions on Image Processing 13, 9Exemplar-based Image Inpainting. (2004), 1200–1212"
"이미지 처리 13, 9 예시 기반 이미지 인페인팅에서의 IEEE 트랜잭션에 의한 영역 채우기 및 객체 제거.(2004), 1200–1212"
"Soheil Darabi, Eli Shechtman, Connelly Barnes, Dan B Goldman, and Pradeep Sen. 2012"
"소힐 다라비, 엘리 셰히트먼, 코넬리 반즈, 댄 B 골드만, 프라딥 센. 2012"
" Image Melding: Combining Inconsistent Images using Patch-based Synthesis. ACM Transactions on Graphics (Proceedings of SIGGRAPH) 31, 4, Article 82 (2012),82:1–82:10 pages"
"이미지 멜딩: 패치 기반 합성을 사용하여 일관성 없는 이미지를 결합합니다.그래픽에 대한 ACM 거래(SIGGRAPH의 절차) 31, 4, 제82조(2012년):1–82:10페이지"
"J. Deng, W"
"J. Deng, W"
" Dong, R. Socher, L"
"동, R. 소처, L"
"-J. Li, K"
"-J. 리, K"
" Li, and L. Fei-Fei"
"리, 그리고 L. 페이페이"
2009. ImageNet: A Large-ScaleHierarchical Image Database
2009년 이미지넷: 대규모 계층적 이미지 데이터베이스
" In CVPR09. Yue Deng, Qionghai Dai, and Zengke Zhang"
"유에덩, 첸가이 다이, 쩡케 장"
2011. Graph Laplace for occluded facecompletion and recognition
"2011년, 폐쇄된 얼굴 완성 및 인식을 위한 그래프 라플라스"
" IEEE Transactions on Image Processing 20, 8 (2011),2329–2338. Iddo Drori, Daniel Cohen-Or, and Hezy Yeshurun"
"IEEE 트랜잭션 on Image Processing 20, 8 (2011), 2329-2338.이도 드로리, 다니엘 코헨 오르, 헤지 예슈룬"
2003. Fragment-based Image Com-pletion
2003년 단편기반 이미지 컴플레이션
" ACM Transactions on Graphics (Proceedings of SIGGRAPH) 22, 3 (2003),303–312. Alexei Efros and Thomas Leung"
"그래픽에 대한 ACM 트랜잭션(SIGGRAPH의 절차) 22, 3(2003),303–312.알렉세이 에프로스와 토마스 렁"
1999. Texture Synthesis by Non-parametric Sampling
비모수 표본 추출에 의한 조직합성
In International Conference on Computer Vision. 1033–1038
컴퓨터 비전에 관한 국제회의.
Alexei A. Efros and William T
알렉세이 A. 에프로스와 윌리엄 T
Freeman. 2001
프리먼 2001
Image Quilting for Texture Synthesis andTransfer. In ACM Transactions on Graphics (Proceedings of SIGGRAPH)
텍스처 합성 및 전송을 위한 이미지 퀼팅입니다.그래픽에 대한 ACM 트랜잭션(SIGGRAPH의 처리)
341–346. Kunihiko Fukushima
341-346 후쿠시마 구니히코
1988. Neocognitron: A hierarchical neural network capable ofvisual pattern recognition
1988. 네오코니트론: 시각적 패턴 인식이 가능한 계층적 신경망
" Neural networks 1, 2 (1988), 119–130. Ian J"
"신경망 1, 2, 1988), 119-130."
" Goodfellow, Jean Pouget-Abadie, Mehdi Mirza, Bing Xu, David Warde-Farley,Sherjil Ozair, Aaron C. Courville, and Yoshua Bengio"
"굿펠로우, 장 푸젯-아바디, 메흐디 미르자, 빙 쉬, 데이비드 워드-팔리, 셰릴 오자어, 애런 C. 쿠르빌, 요슈아 벵지오"
2014. Generative AdversarialNets
"2014년, 생성적 대립적 네트"
In Conference on Neural Information Processing Systems. 2672–2680
신경정보처리시스템에 관한 회의제2672~2680조
James Hays and Alexei A. Efros
제임스 헤이스와 알렉세이 A. 에프로스
2007. Scene Completion Using Millions of Photographs
수백만 장의 사진을 이용한 장면 완성
"ACM Transactions on Graphics (Proceedings of SIGGRAPH) 26, 3, Article 4 (2007). Kaiming He and Jian Sun"
"그래픽에 대한 ACM 거래(SIGGRAPH의 절차) 26, 3, 제4조(2007).카이밍 헤이와 지안 선"
2012. Statistics of Patch O(cid:29)sets for Image Completion
2012년 이미지 완성을 위한 패치 O (cid : 29) 세트의 통계
InEuropean Conference on Computer Vision. 16–29
유럽 컴퓨터 비전 회의 16~29
"Jia-Bin Huang, Sing Bing Kang, Narendra Ahuja, and Johannes Kopf. 2014"
"지아빈황, 싱빙강, 나렌드라 아후자, 요하네스 콥프. 2014"
" Image Com-pletion Using Planar Structure Guidance. ACM Transactions on Graphics (Proceedingsof SIGGRAPH) 33, 4, Article 129 (2014), 10 pages"
"평면 구조 지침을 사용하는 이미지 컴플레이션.그래픽에 대한 ACM 거래(SIGGRAPH의 처리) 33, 4, 제129조(2014년), 10페이지"
Sergey Io(cid:29)e and Christian Szegedy. 2015
Sergey Io (cid : 29)e와 Christian Szegedy. 2015
Batch Normalization: Accelerating DeepNetwork Training by Reducing Internal Covariate Shift. In International Conferenceon Machine Learning
배치 정규화 : 내부 공변량 이동을 줄임으로써 DeepNetwork 훈련 가속화.국제회의 기계학습에서
"Phillip Isola, Jun-Yan Zhu, Tinghui Zhou, and Alexei A Efros. 2017"
"필립 이솔라, 준얀 주, 팅후이 주, 알렉세이 A 에프로스. 2017년"
Image-to-ImageTranslation with Conditional Adversarial Networks. (2017)
조건부 상대적 네트워크와의 이미지 대 이미지 변환(2017)
Jiaya Jia and Chi-Keung Tang. 2003
지야지아와 치경당 2003년
" Image repairing: robust image synthesis by adaptiveND tensor voting. In IEEE Conference on Computer Vision and Pattern Recognition,Vol"
"이미지 수리 : 적응형 ND 텐서 투표에의 한 강력한 이미지 합성.IEEE 컨퍼런스에서 컴퓨터 비전과 패턴 인식, Vol"
1. 643–650
1. 643–650
"Rolf Köhler, Christian Schuler, Bernhard Schölkopf, and Stefan Harmeling. 2014"
"롤프 쾰러, 크리스찬 슐러, 베른하르트 ö코프, 스테판 하멜링. 2014년"
Mask-speci(cid:27)c inpainting with deep neural networks. In German Conference on PatternRecognition
깊은 신경망을 가진 마스크-스페시(cid:27)c.독일 문양인식회의
"Johannes Kopf, Wolf Kienzle, Steven Drucker, and Sing Bing Kang. 2012"
"요하네스 코프, 울프 키엔즐, 스티븐 드러커, 싱빙강."
" QualityPrediction for Image Completion. ACM Transactions on Graphics (Proceedings ofSIGGRAPH Asia) 31, 6, Article 131 (2012), 8 pages"
"이미지 완료에 대한 품질 예측입니다.그래픽에 대한 ACM 거래(SIGGRAPH 아시아의 절차) 31, 6, 제131조(2012), 8페이지"
"Vivek Kwatra, Irfan Essa, Aaron Bobick, and Nipun Kwatra. 2005"
"2005년 비벡 콰트라, 이르판 에사, 애런 보빅, 니푼 콰트라."
" Texture Optimiza-tion for Example-based Synthesis. ACM Transactions on Graphics (Proceedings ofSIGGRAPH) 24, 3 (July 2005), 795–802"
"예제 기반 합성을 위한 텍스처 옵티미자션.그래픽에 대한 ACM 트랜잭션(SIGGRAPH의 절차) 24, 3 (2005년 7월), 795-802"
"Vivek Kwatra, Arno Schödl, Irfan Essa, Greg Turk, and Aaron Bobick. 2003"
"2003년 비벡 콰트라, 아르노 ö들, 이르판 에사, 그렉 터크, 애런 보빅."
" GraphcutTextures: Image and Video Synthesis Using Graph Cuts. ACM Transactions onGraphics (Proceedings of SIGGRAPH) 22, 3 (July 2003), 277–286"
"그래프 텍스처: 그래프 컷을 사용한 이미지 및 비디오 합성.그래픽에 대한 ACM 거래(SIGGRAPH의 절차) 22, 3 (2003년 7월), 277-286"
"Yann LeCun, Bernhard Boser, John S Denker, Donnie Henderson, Richard E Howard,Wayne Hubbard, and Lawrence D Jackel. 1989"
"Yann LeCun, Bernhard Boser, John S Denker, Donnie Henderson, Richard E Howard, Wayne Hubbard 및 Lawrence D Jackel.1989년"
" Backpropagation applied to hand-written zip code recognition. Neural computation 1, 4 (1989), 541–551"
"수기 우편번호 인식에 적용된 역전파.신경 계산 1, 4 (1989), 541-551"
"ACM Transactions on Graphics, Vol. 36, No"
"그래픽에 대한 ACM 거래, Vol. 36, No"
" 4, Article 107. Publication date: July 2017"
제107조 출판일: 2017년 7월
"Anat Levin, Assaf Zomet, and Yair Weiss. 2003"
"아나트 레빈, 아사프 조메트, 야이르 와이스. 2003년"
Learning How to Inpaint from GlobalImage Statistics. In International Conference on Computer Vision
글로벌 이미지 통계에서 인페인팅하는 방법 학습.컴퓨터 비전에 관한 국제회의
" 305–312. Rongjian Li, Wenlu Zhang, Heung-Il Suk, Li Wang, Jiang Li, Dinggang Shen, andShuiwang Ji"
"305-312. 룽젠 리, 원루 장, 흥일석, 리왕, 장리, 딩강 선, 슈이왕 지"
2014. Deep learning based imaging data completion for improvedbrain disease diagnosis
2014. 뇌질환 진단 개선을 위한 딥러닝 기반 영상데이터 완성
" In International Conference on Medical Image Computingand Computer-Assisted Intervention. Springer, 305–312"
"국제 의료 영상 컴퓨팅 및 컴퓨터 지원 중재 회의에서.스프링거, 305-312"
"Ziwei Liu, Ping Luo, Xiaogang Wang, and Xiaoou Tang. 2015"
"지웨이 류, 핑루오, 샤오강 왕, 샤오우 당. 2015년"
Deep Learning FaceAttributes in the Wild. In International Conference on Computer Vision
야생에서의 심층학습 얼굴속성
"Jonathan Long, Evan Shelhamer, and Trevor Darrell. 2015"
"조나단 롱, 에반 셸하머, 트레버 대럴. 2015년"
Fully convolutional networksfor semantic segmentation. In IEEE Conference on Computer Vision and PatternRecognition
의미분할을 위한 완전한 컨볼루션 네트워크컴퓨터 비전과 패턴 인식에 관한 IEEE 컨퍼런스
"Umar Mohammed, Simon JD Prince, and Jan Kautz. 2009"
"우마르 모하메드, 사이먼 JD 프린스, 얀 카우츠. 2009"
" Visio-lization: generatingnovel facial images. ACM Transactions on Graphics (Proceedings of SIGGRAPH) 28, 3(2009), 57"
"Visio-lization : 새로운 얼굴 이미지를 생성합니다.그래픽에 대한 ACM 트랜잭션(SIGGRAPH의 절차) 28, 3(2009), 57"
Vinod Nair and Geo(cid:29)rey E Hinton. 2010
Vinod Nair와 Geo (cid : 29)rey E Hinton.
Recti(cid:27)ed linear units improve restrictedboltzmann machines. In International Conference on Machine Learning
Recti (cid : 27) 선형 단위는 제한된 볼츠만 기계를 개선합니다.기계학습에 관한 국제회의
" 807–814. Deepak Pathak, Philipp Krähenbühl, Je(cid:29) Donahue, Trevor Darrell, and Alexei Efros"
"Deepak Pathak, Philipp Krähenbühl, Je (cid : 29) Donahue, Trevor Darrell 및 Alexei Efros"
2016. Context Encoders: Feature Learning by Inpainting
2016 컨텍스트 인코더 : 인페인팅에 의한 특징 학습
" In IEEE Conference onComputer Vision and Pattern Recognition. Darko Pavić, Volker Schönefeld, and Leif Kobbelt"
"컴퓨터 비전 및 패턴 인식에 관한 IEEE 컨퍼런스에서.다코 파비, 볼커 ö네펠트, 라이프 코벨트"
2006. Interactive image completionwith perspective correction
"2006년, 원근법 보정을 통한 인터랙티브 영상 완성"
" The Visual Computer 22, 9 (2006), 671–681. Patrick Pérez, Michel Gangnet, and Andrew Blake"
"비주얼 컴퓨터 22, 9(2006), 671–681. 패트릭 페레즈, 미셸 갱넷, 앤드류 블레이크"
2003. Poisson Image Editing
2003년 포아송 이미지 편집
" ACMTransactions on Graphics (Proceedings of SIGGRAPH) 22, 3 (July 2003), 313–318. Alec Radford, Luke Metz, and Soumith Chintala"
"그래픽에 대한 ACMTransactions on Graphics (SIGGRAPH의 절차) 22, 3 (2003년 7월), 313-318.알렉 래드포드, 루크 메츠, 그리고 수미스 킨탈라"
2016. Unsupervised RepresentationLearning with Deep Convolutional Generative Adversarial Networks
"2016, 심층 컨볼루션 생성적 역방향 네트워크를 이용한 무감독 표현 학습"
In Interna-tional Conference on Learning Representations. Radim Šára Radim Tyleček
학습 표현에 관한 국제회의에서 라딤 라딤 타일렉
2013. Spatial Pattern Templates for Recognition of Objectswith Regular Structure
2013년. 규칙적인 구조를 가진 물체 인식을 위한 공간 패턴 템플릿
" In German Conference on Pattern Recognition. Saarbrucken,Germany"
독일 문양인식회의에서. 독일 사브루켄
"Jimmy SJ Ren, Li Xu, Qiong Yan, and Wenxiu Sun. 2015"
"지미 SJ 렌, 리 쉬, 첸연, 웬시우 선. 2015년"
Shepard Convolutional NeuralNetworks. In Conference on Neural Information Processing Systems
신경정보처리시스템에 관한 연구
D. E
디에이
" Rumelhart, G. E"
"루멜하트, G. E"
" Hinton, and R. J"
"힌튼, R.J"
Williams. 1986
"윌리엄스, 1986년"
Learning representations byback-propagating errors. In Nature
학습 표상은 역전파 오류를 반영한다.
"Tim Salimans, Ian Goodfellow, Wojciech Zaremba, Vicki Cheung, Alec Radford, andXi Chen. 2016"
"팀 살리만스, 이안 굿펠로우, 워지치치 자렘바, 비키 청, 알렉 래드포드, 시첸. 2016년"
Improved techniques for training gans. In Conference on NeuralInformation Processing Systems
GAN 훈련 기술의 개선. 신경정보 처리시스템에 관한 회의
"Denis Simakov, Yaron Caspi, Eli Shechtman, and Michal Irani. 2008"
"데니스 시마코프, 야론 카스피, 일라이 셰히트만, 미할 이란어. 2008"
Summarizingvisual data using bidirectional similarity. In IEEE Conference on Computer Vision andPattern Recognition
양방향 유사성을 사용하여 시각 데이터를 요약합니다.컴퓨터 비전과 패턴 인식에 관한 IEEE 컨퍼런스
" 1–8. Jian Sun, Lu Yuan, Jiaya Jia, and Heung-Yeung Shum"
"1-8. 지안선, 루위안, 지야자, 흥영섬"
2005. Image Completion withStructure Propagation
2005년 구조 전파를 통한 영상 완성
" ACM Transactions on Graphics (Proceedings of SIGGRAPH)24, 3 (July 2005), 861–868. DOI:https://doi"
"그래픽에 대한 ACM 트랜잭션 (SIGGRAPH의 절차)24, 3 (2005년 7월), 861-868."
org/10.1145/1073204.1073274Alexandru Telea. 2004
org/10.1145/1073204.1073274Alexandru Telea. 2004
" An Image Inpainting Technique Based on the Fast MarchingMethod. Journal of Graphics Tools 9, 1 (2004), 23–34"
"빠른 마칭 방식을 기반으로 한 이미지 인페인팅 기법그래픽 도구 저널 9, 1 (2004), 23-34"
"Yonatan Wexler, Eli Shechtman, and Michal Irani. 2007"
"요나탄 슬러, 엘리 셰흐트만, 미할 이란어. 2007년"
" Space-Time Completion ofVideo. IEEE Transactions on Pattern Analysis and Machine Intelligence 29, 3 (2007),463–476"
"비디오의 시공간 완성.패턴분석 및 기계정보에 관한 IEEE 거래 29, 3 (2007),463-476"
"Oliver Whyte, Josef Sivic, and Andrew Zisserman. 2009"
"올리버 왜트, 요제프 시빅, 앤드류 지서먼. 2009년"
Get Out of my Picture! Internet-based Inpainting. In British Machine Vision Conference
내 그림에서 나가! 인터넷 기반 인페인팅. 영국 기계 비전 컨퍼런스에서
"Junyuan Xie, Linli Xu, and Enhong Chen. 2012"
"주뉴안 시, 린리 쉬, 엔홍첸. 2012"
Image Denoising and Inpainting withDeep Neural Networks. In Conference on Neural Information Processing Systems
Deep Neural Networks로 이미지 잡음 제거 및 인페인팅.신경정보처리시스템에 관한 회의
"341–349. Chao Yang, Xin Lu, Zhe Lin, Eli Shechtman, Oliver Wang, and Hao Li"
"차오양, 신루, 제린, 일라이 셰히트만, 올리버 왕, 하오리"
2017. High-Resolution Image Inpainting using Multi-Scale Neural Patch Synthesis
2017년 다차원 신경 패치 합성을 이용한 고해상도 이미지 인페인팅
In IEEEConference on Computer Vision and Pattern Recognition. Fisher Yu and Vladlen Koltun
컴퓨터 비전과 패턴 인식에 관한 IEEEConference 연구
2016. Multi-Scale Context Aggregation by DilatedConvolutions
2016년 확장된 컨볼루션을 이용한 다단계 컨텍스트 집합
In International Conference on Learning Representations. Matthew D
국제학술대회에서의 대표성
Zeiler. 2012
제일러 2012
ADADELTA: An Adaptive Learning Rate Method. CoRRabs/1212.5701 (2012)
ADADELTA : 적응 학습 속도 방법. CoRRabs/1212.5701 (2012)
"Bolei Zhou, Aditya Khosla, Àgata Lapedriza, Antonio Torralba, and Aude Oliva. 2016"
"볼레이 저우, 아디타 코슬라, 라가타 라페드리자, 안토니오 토랄바, 오드 올리바. 2016년"
Places: An Image Database for Deep Scene Understanding. CoRR abs/1610.02055(2016)
장소: 심층 장면 이해를 위한 이미지 데이터베이스. CoRR abs/1610.02055(2016)
'딥러닝 > 논문리뷰' 카테고리의 다른 글
A Robust Predictive Model for Stock Price Prediction Using Deep Learning and Natural Language Processing 감성분석을 이용한 주가 예측 (0) | 2021.01.12 |
---|---|
[논문 코드 구현]global local image completion (0) | 2020.11.23 |
global local - 3.1 Convolutional Neural Networks (0) | 2020.11.05 |
[OCR] TLGAN 적은 양의 데이터를 필요로 하는 텍스트 위치 결정 모델 (0) | 2020.11.03 |
attention is all you need 한글 번역 (0) | 2020.11.02 |
댓글